Ayush Mehta’s Journey from Engineer to Data Scientist
Ayush Mehta's journey from an electronics engineer to a data scientist showcases the power of continuous learning and adaptability. His transition, fueled by certifications and hands-on projects, highlights how passion and persistence can lead to success in the dynamic field of data science.
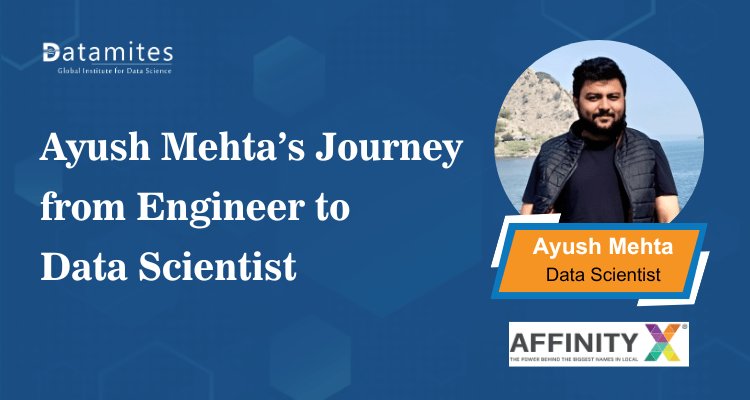
The path to becoming a Data Scientist is rarely a straight line. For many, it’s a journey filled with exploration, adaptation, and continuous learning. One such inspiring journey is that of Ayush Mehta, who successfully transitioned from an electronics engineering background to a Data Scientist role at AffinityX, a prominent marketing and advertising solutions company.
Ayush Mehta’s journey is a testament to how perseverance, structured learning, and adaptability can lead to a successful career in data science. With a background in electronics and instrumentation, he transitioned into data science by earning relevant certifications, working on hands-on projects, and exploring diverse roles in the field. His story highlights how, with the right guidance and mindset, anyone can break into data science.
Ayush Mehta’s Path to Data Science: A Success Story with DataMites
His journey reflects a seamless transition fueled by passion, upskilling, and the right mentorship from DataMites.
1. Can you tell us a little about your background and journey into data science?
I did my graduation in electronics and instrumentation and later pursued a master’s in embedded systems and IoT from the UK. However, the COVID pandemic disrupted my career path. I decided to explore the data field and started learning about data analysis and algorithms. After gaining confidence, I enrolled in structured courses from platforms like DataMites Institute. This helped me land my first job as a data analyst. Over time, I worked my way up to a data scientist position, learning everything from data engineering to predictive modeling along the way.
2. How did DataMites help you in your career transformation?
The structure and curriculum offered by DataMites were really helpful. The courses were well-organized, and the hands-on projects provided a real-world context to apply what I was learning. I believe more than 50% of my learning came from the projects, including the capstone projects. They helped me gain a lot of practical exposure, which was instrumental in my career growth.
3. Was transitioning from a developer role to a data scientist role challenging for you?
It wasn’t as tough as one might expect. The key to transitioning smoothly was continuous learning. When I started as a developer, I could have stayed there, but I always wanted to grow. I kept learning new things and kept up with the latest technologies. Completing my diploma in machine learning and AI helped validate my skills and gave me more confidence.
4. How important is continuous learning in the field of data science?
Continuous learning is critical. You cannot afford to stay stagnant. The field of data science is constantly evolving, and the tools and techniques you used yesterday may not be applicable tomorrow. By keeping up with new developments, attending courses, and getting certifications, you’re always ready for the next opportunity.
5. Do you believe that data visualization is a significant part of a data scientist’s job?
Yes, absolutely. Visualization is essential because it allows you to present data in a way that is easy to understand and interpret. For me, the process of storytelling with data was something I found incredibly rewarding. I was able to not only present raw data but also craft visual reports that made complex data insights accessible.
6. How important is it for data scientists and analysts to understand databases?
Databases are the foundation of data science. If you want to work in any data-driven industry, knowing how to work with databases is essential. While algorithms and coding are important, you cannot extract insights without understanding how to store, retrieve, and manipulate data effectively. A strong understanding of SQL, database management, and data pipelines will always give you an edge.
7. Can you elaborate on the importance of Excel in data analysis?
Excel can be a powerful tool for data analysis, especially when dealing with small datasets or when you need quick analysis. However, in larger organizations or with more complex datasets, you’ll likely need to rely on specialized tools like SQL, Python, or visualization software. So, Excel is useful but not always sufficient on its own.
8. How did your background in electronics help you transition into data science?
My background in electronics and IoT helped me build problem-solving skills. Even though I was initially focused on hardware and embedded systems, I was always fascinated by how AI and machine learning could be integrated into various systems. That curiosity eventually led me to pursue data science.
9. How important is it to specialize in one area within data science?
Specialization can be beneficial, but having a broad knowledge base also helps. Ideally, you should focus on one core skill—whether it’s machine learning, data engineering, or data visualization—but at the same time, it’s helpful to have some knowledge of the other areas. Being a well-rounded data scientist gives you more opportunities and makes you adaptable to different roles.
10. How crucial is it to learn tools like SQL, databases, and machine learning algorithms for aspiring data scientists?
SQL and databases form the backbone of data science. Machine learning algorithms are the core of predictive modeling and analysis. A strong understanding of these concepts will make you more competitive in the job market and more effective in your role. It’s essential to master these foundational tools.
11. Can you explain the concept of "full-stack data science"?
Full-stack data science refers to having a broad skill set that includes data collection, data engineering, machine learning, and visualization. Many companies, especially startups and smaller firms, may prefer hiring one person who can handle all aspects of the data process rather than multiple specialists. So, full-stack data scientists are highly versatile and valuable.
12. Do you believe that Gen AI is reshaping the data science field?
Yes, Gen AI is changing the game. While traditional data science techniques are still important, Gen AI has enabled automation and more efficient data processing. Being familiar with Gen AI tools gives you an edge in performing tasks like data pre-processing and visualization more efficiently.
13. How do you suggest beginners approach learning data science?
Start with the basics. Learn SQL and database management first. Once you’re comfortable with that, move on to visualization tools like Tableau or Power BI. Then, focus on learning machine learning algorithms and data preprocessing techniques. The more hands-on projects you complete, the better you will understand how to apply these tools in real-world situations.
14. How do soft skills factor into your role as a data scientist?
Communication skills are very important. As a data scientist, it's essential to communicate your insights in a clear and simple manner that can be easily understood by non-technical stakeholders. Storytelling with data is a key part of the role, and being able to communicate insights effectively is critical.
15. Do you feel that your work experience prepared you for the role of a data scientist?
Absolutely. The hands-on experience I gained while working on various projects, learning different tools, and solving real-world problems helped me immensely. My experience with data engineering, machine learning, and visualization gave me a solid foundation for my current role as a data scientist.
16. What was the biggest challenge during your transition from an intern to a data scientist?
The biggest challenge was learning to manage different tasks across various stages of the data science pipeline. For instance, when I first worked with Tableau, I had no experience with the tool, so I had to quickly adapt and learn how to use it effectively. Each transition had a learning curve, but that’s what made the journey worthwhile.
17. What role do projects play in learning data science?
Projects are key to applying the concepts you learn. They give you a platform to experiment with different tools and techniques, allowing you to refine your skills. Practical projects provide valuable learning experiences and help your resume catch the attention of potential employers.
18. Do you think certifications are necessary for a career in data science?
Certifications validate your skills and knowledge. While hands-on experience is important, certifications from credible institutions like DataMites provide a solid foundation and demonstrate your commitment to the field. They can open doors to job opportunities and are particularly helpful when you're just starting out.
19. What advice do you have for aspiring data scientists?
Stay curious, and always be willing to learn. Data science is an ever-evolving domain, requiring professionals to stay updated with emerging tools and methodologies. Don’t be afraid to take on challenging projects and try new things. Most importantly, never stop learning.
20. Where do you see yourself in the next few years in terms of your career in data science?
I see myself continuing to grow in the data science field, especially focusing on AI applications and predictive analytics. I also aim to take on more leadership roles, guiding teams of data scientists and driving data-driven decision-making in organizations.
Refer these articles:
- Mechanical Engineer to Data Scientist: The Journey of Vishwas
- Non-Tech to Data Science: Dinesh’s Inspiring Path
- Becoming a Data Scientist: Atif Ansari’s Inspiring Career Leap
Ayush Mehta's Data Science Journey: Valuable Insights and Lessons Learned
In this conversation, he shares key experiences, challenges, and advice that have shaped his career in the dynamic field of data science.
- Background in Electronics & Instrumentation, transitioned to Data Science during COVID-19.
- Worked in analytics to confirm interest in data science.
- Started as a Data Analyst, focusing on data visualization with Tableau, Excel, Power BI.
- Promoted to Data Scientist after gaining experience in AI applications.
- Completed a Machine Learning & AI diploma, pursuing a Master’s degree.
- Emphasized the importance of strong database knowledge in data science roles.
- Excel remains useful for data organization and analysis.
- Aspiring data scientists should learn SQL, data visualization, and machine learning basics.
- Faced challenges transitioning from intern to Data Scientist, adapted quickly to new tools.
- Creativity is crucial in data visualization and reporting.
- Hands-on experience through projects built confidence and practical skills.
- Versatile skills in SQL, data engineering, machine learning, and visualization are highly valued.
- Small and medium businesses need professionals with broad data science skills, including use of Gen AI tools.
Refer these articles:
Ayush Mehta's journey is a testament to the power of adaptability, continuous learning, and hands-on experience. His ability to transition from a developer role to a data scientist illustrates that anyone, regardless of their background, can break into the field of data science with the right approach. The key takeaway from Ayush’s story is that no matter where you start, with passion, persistence, and the right tools, you can reach new heights in the exciting world of data science.
If you’re considering a career shift into data science, Ayush Mehta’s journey proves that now is the perfect time to make the leap. With the global data science platform market valued at USD 96.25 billion in 2023 and expected to grow at a 26.0% CAGR from 2024 to 2030 as per Grand View Research, the field is booming with opportunities. Ayush recognized this trend early and chose to upskill through a program that emphasized hands-on learning, real-time internships, and strong placement support—key elements for success in a competitive industry. Enrolling in a data science course in Pune, Bangalore, Hyderabad, Chennai, Mumbai, and Delhi can be the first step toward building a strong tech career.
Ayush enrolled with DataMites Institute, a leading training provider in Data Science, AI, Machine Learning, Python Development, and Data Analytics. Accredited by IABAC and NASSCOM FutureSkills, DataMites gave him access to expert-led instruction, real-world projects, and robust placement assistance that helped him smoothly transition into the data field.
With flexible learning options—including Offline Data Science training in Pune, Bangalore, Hyderabad, Chennai, Ahmedabad, Coimbatore, and Mumbai—Ayush was able to choose a format that suited his schedule and learning style. DataMites' industry-relevant curriculum empowered him, like many others, to successfully pivot into a data-driven career, regardless of his original background in electronics and instrumentation.