From Non-Tech to Tech: Sowmiya’s Path to AI-ML Engineer
The inspiring story of Sowmiya, who transitioned from a non-technical background into the world of Artificial Intelligence and Machine Learning. Despite initial challenges, she demonstrated resilience, a growth mindset, and a commitment to continuous learning.
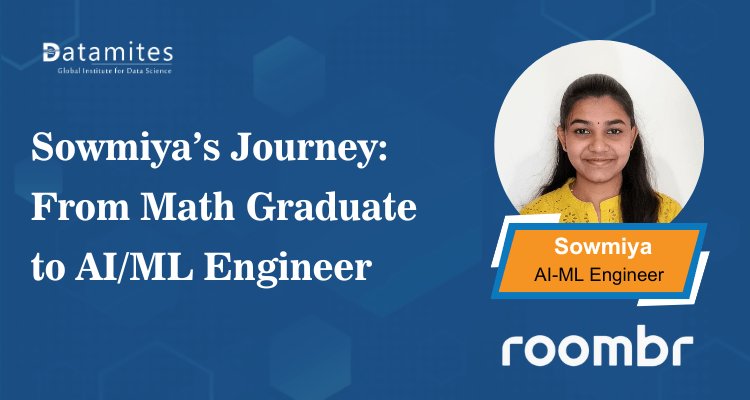
Sowmiya’s journey is nothing short of remarkable. With no technical background in coding or data science, she dared to rewrite her career story—armed only with resilience, curiosity, and a dream to break into tech. In an industry where competition is fierce and change is constant, Sowmiya not only made the leap but soared. Her inspiring transformation proves that with the right mindset and support, anyone can pivot to a future powered by innovation.
Sowmiya’s Interview Experience, Learning Journey, and Career Advice
Curious how she did it? Let’s dive into Sowmiya’s story of reinvention and rise in the world of Artificial Intelligence and Machine Learning.
Q1: Sowmiya, can you please introduce yourself and share your academic background?
Hi everyone, I’m Sowmiya Lakshmanin. I hold a Bachelor's and Master's degree in Mathematics, with my postgraduate studies completed at Sastra University. I’ve always been passionate about numbers and logical reasoning, which eventually led me to discover the vast opportunities in the field of data science. I am now a certified data science professional from DataMites and thrilled to be a part of Rubixe.
Q2: How did you make the connection between mathematics and data science?
There’s a natural overlap between mathematics and data science, especially in areas like statistics, modeling, and machine learning. My academic foundation helped me understand the theoretical aspects, which made data science a logical next step. I realized this connection during my exploration of career options and decided to transition into IT through DataMites' structured program.
Q3: Coming from a non-coding background, was learning Python a challenge for you?
Initially, I had minimal exposure to Python. But with consistent effort and practice, I became comfortable within a few months. Surprisingly, Python turned out to be a remarkably intuitive and beginner-friendly language, especially when compared to other programming languages. It took me around 3–4 weeks to become proficient enough to apply it in data science contexts.
Q4: How did your mathematics background help you with statistics and machine learning?
My academic training provided me with a solid understanding of statistics, so I didn’t face major difficulties there. As for machine learning, although it was a new domain for me, the mathematical underpinnings made it easier to grasp the concepts. With dedicated learning, I was able to build a strong understanding of ML within about 2 months.
Q5: How long did it take to complete the course and become industry-ready?
The entire journey—from learning Python and statistics to completing projects and preparing for interviews—took about 9 months. I spent the initial 4 months attending live sessions and understanding the core concepts. Then, I dedicated another few months to capstone and live projects, followed by 1–2 months of focused interview preparation.
Q6: What kind of projects did you work on during the course?
I worked on various capstone projects involving classification, regression, and clustering. My most significant project was a live client assignment on time series forecasting, focused on predicting the availability of sports spare parts—a classic example of inventory management. I completed this project individually, which boosted my confidence significantly.
Q7: When did the placement support begin, and how was the experience?
The placement support from DataMites started in October 2023, after my internship phase. They facilitated interviews and provided continuous support through resume building, interview scheduling, and feedback sessions. I appeared for two interviews, and although I didn't clear the final stage of the second one, it gave me valuable insights into industry expectations.
Q8: How did you prepare for job interviews after completing the course?
After completing the live project, I spent an additional 1–2 months refining my technical and soft skills, mock interviews, and reviewing common data science interview questions. The goal was to become comfortable discussing projects, justifying model choices, and handling real-world business scenarios.
Q9: Could you share your interview experience? How many rounds were there?
Yes, absolutely! The interview process included three rounds in total. The first round was an assessment focused on basic Python programming and statistics concepts. It tested fundamental technical knowledge, which is expected from most candidates, especially freshers.
Q10: Were there any machine learning questions involved?
It really depended on the company and the role. In one interview, there weren’t any ML-specific questions. But with another company, yes—I was asked questions related to machine learning, particularly on ensemble techniques like bagging and boosting. Since I was confident in these areas, I could explain them well.
Q11: Does doing an internship help in getting a job in AI/ML?
Absolutely. An internship provides a smooth transition into the workforce. When candidates say they’ve only learned the concepts, it may not be enough. But when they can show real-world experience through internships or live projects, it increases their credibility. Recruiters are interested in the kind of projects you worked on, challenges you overcame, and your role in the team. Practicing is crucial, and internships offer hands-on learning and valuable talking points during interviews.
Q12: How long does it take to complete the DataMites capstone and client projects and secure a job?
On average, it takes about 2 to 3 months to complete all capstone and client projects. However, job placement varies. Some candidates secure jobs during the project phase itself, while others land roles within 2–3 months post-completion.
Q13: How is the work-life balance for a data scientist?
Work-life balance for data scientists is similar to that of other IT professionals. However, it can sometimes be more unpredictable. This is because data science projects, especially those involving machine learning models, come with a level of uncertainty—the model might not always perform as expected. This unpredictability can sometimes lead to more intense project timelines. That said, it largely depends on the organization and the nature of the project.
Q14: Can a basic data science curriculum help crack interviews if companies are now focusing on Generative AI (GenAI)?
Yes, a strong foundation in data science fundamentals—including Python, machine learning, neural networks, and statistics—is still highly valuable. While GenAI is gaining traction, many companies are open to training candidates in specific tools and frameworks after hiring. DataMites AI Engineer course is recommended for those who want to specialize in GenAI, LLMs, and NLP.
Q15: What would be your message to other non-coders or math graduates wanting to pursue data science?
Don’t let your non-programming background hold you back. If you have a passion for problem-solving and data, this field has a place for you. Choose a structured program, stay consistent, and practice regularly. Python and machine learning are learnable skills—even if you’re starting from scratch. My journey is proof that you can bridge the gap with the right support and mindset.
Refer these below articles:
- Nikhil Maurya’s NLP Career Path
- How Bhavya Made a Successful Leap into Artificial Intelligence
- From Engineer to ML Engineer: Rahul Thota’s Inspiring Journey
Key Findings from the Sowmiya Career Talk
Sowmiya’s journey from a non-tech background to becoming an AI-ML engineer is a testament to determination and continuous learning. Her story offers valuable insights on career transition, self-motivation, and the power of upskilling.
1. Work-Life Balance in Data Science
Sowmiya shared that while it’s still early in her career to make a conclusive statement, the general work-life balance in her current company is manageable.
2. Timeline to Complete Projects and Land a Job
According to Sowmiya:
- It took her 2 to 3 months to complete capstone and client projects.
- Job placement timelines vary—25-30% of students get placed during or immediately after their project phase.
3. Curriculum and Industry Relevance
There was a concern about whether basic curriculum helps in a GenAI-focused market:
- The CDS course is frequently updated, with major syllabus changes over the years.
- GenAI, LLMs, and advanced NLP are covered in the AI Engineering course, a 1-year program.
- Recruiters prioritize strong fundamentals in ML, statistics, and Python, and are willing to train new hires in specialized domains like GenAI.
4. Hiring Focus
- Employers prioritize strong fundamentals (ML, neural networks, Python, statistics).
- GenAI can be learned on the job (e.g., Sowmiya being trained post-placement).
5. Job Placement Timeline
- 25–30% of students secure jobs during or shortly after their project phase.
- Remaining candidates usually take a few additional months, depending on effort and consistency.
6. Support for Career Gaps
- Alumni can access free mentoring and revision sessions, even years after completing the course.
- Career gaps do not necessarily hinder re-entry with the right preparation.
Read these below articles:
- How is the demand for Artificial Intelligence shaping up in Noida?
- The Growing Demand for Artificial Intelligence in Chandigarh
- How Much is Artificial Intelligence in Demand in Nagpur?
Sowmiya’s story is a testament to persistence and structured learning. With no prior IT experience, she secured a job in AI by mastering the basics, staying confident in interviews, and continuously upgrading her skills.
The global artificial intelligence market surpassed $184 billion USD in 2024, marking a significant increase of nearly $50 billion compared to 2023. This remarkable growth is projected to continue, with the market expected to exceed $826 billion USD by 2030 (Statista). With an annual growth rate of approximately 35%, particularly in AI and Data Science, the field offers vast opportunities for individuals who are committed to continuous learning and practical application. Whether you're a student, career switcher, or job seeker, success lies in leveraging internships, hands-on projects, and maintaining strong self-discipline.
DataMites offers a wide range of expertly crafted courses in artificial intelligence and machine learning, catering to learners across all proficiency levels from complete beginners to seasoned professionals. These programs are thoughtfully structured to combine in-depth theoretical knowledge with hands-on practical experience, often including live projects and internship opportunities to ensure real-world applicability. As an authorized training partner of IABAC and NASSCOM FutureSkills, DataMites provides globally recognized certifications that add value to your professional profile.
The Artificial Intelligence Course in Bangalore is a comprehensive, industry-aligned training program designed to equip learners with the skills and knowledge needed to excel in the rapidly evolving field of AI. Whether you are a beginner or a working professional looking to upskill, the course covers everything from the fundamentals of artificial intelligence to advanced topics like machine learning, deep learning, natural language processing (NLP), and computer vision.
The Artificial Intelligence Training in Hyderabad by DataMites is designed to cater to diverse learning preferences with flexible options including classroom training, live online sessions, and self-paced learning. These formats allow learners to choose the mode that best fits their schedule and learning style. The AI training participants gain access to strong career support, featuring personalized resume building, mock interview sessions, and regular job opportunity updates. This makes the program an excellent launchpad for individuals aiming to begin or advance their careers in the ever-growing field of artificial intelligence.