Non-Tech to Data Science: Dinesh’s Inspiring Path
Dinesh’s transition from a non-technical background to a successful data science career showcases the power of determination and continuous learning. Through DataMites’ comprehensive training, he gained the skills and confidence to thrive in this dynamic field.
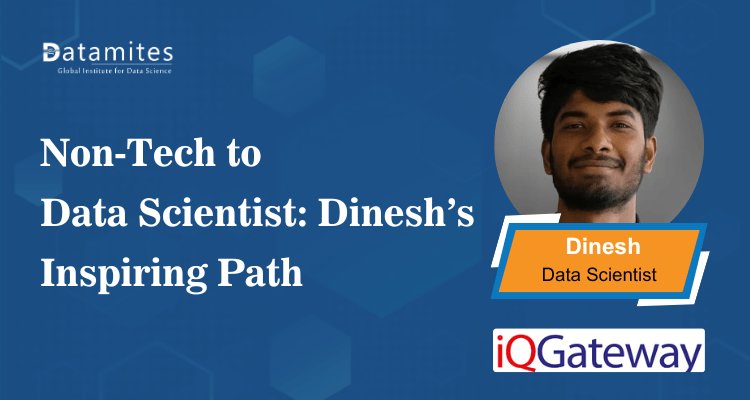
Breaking into the field of data science can seem overwhelming, especially for individuals without a formal technical background. However, with the right approach, continuous learning, and strategic preparation, it's absolutely achievable. In this blog, we explore a detailed account of a data science interview experience with IQ Gateway, shared by a successful candidate who was trained by DataMites. This comprehensive interview breakdown includes the number of rounds, the types of assessments, key advice, and practical tips to help aspiring data scientists prepare effectively.
Dinesh's Data Science Journey: Charting Success with DataMites
Dinesh's journey into data science with DataMites has been one of growth, learning, and transforming his career through hands-on training and real-world experience.
Q1: Can you tell us a bit about your background and how you started your journey towards Data Science?
I have a degree in Mechanical Engineering, which I completed in 2020. I worked for about 1.5 years as a supervisor in inventory management at a CNC-based company. Coming from a non-technical background, I decided to shift my career toward Data Science. I enrolled in a course with DataMites Institute around September, got certified, and later secured a position at a company in Bangalore. I believe my mechanical background helped foster innovative thinking, which has been valuable in transitioning to the IT field.
Q2: How did you prepare for your interviews?
I didn't focus as much on traditional practice but instead, focused on doing real projects. I believe that hands-on experience is crucial. I worked on a few machine learning and deep learning projects, which gave me the practical knowledge required for interviews.
Q3: How many interview rounds did you go through?
I had six rounds in total. The first four rounds were quite similar, focusing on the technical side, with the last two rounds being more related to discussions and final evaluations.
Q4: Can you walk us through the six rounds of interviews you experienced?
Sure! Here’s a breakdown of the six rounds:
- First Round: Mindset and Aptitude Testing
- Second Round: A technical project involving Machine Learning, Deep Learning, and NLP where you are expected to deploy the project
- Third Round: Communication and Behavioral Interview
- Fourth Round: Technical Interview 1
- Fifth Round: Technical Interview 2
- Sixth Round: A discussion with senior members (like directors and VPs) about your technical and professional approach.
Q: Can you elaborate on the first round and what it involved?
The first round was primarily about testing my mindset and aptitude. This included psychometric tests and repeated questions to check consistency in my answers. It was designed to assess how well I think and react under pressure.
Q: What was the second round like?
The second round was a hands-on technical project. They gave me a choice of three different types of projects in Machine Learning, Deep Learning, and NLP. I had about one or two days to complete the task and deploy it. It was an open-book test, but the key was demonstrating a clear understanding of the concepts and the ability to deploy the model efficiently.
Q: What was assessed in the third round of the interview?
The third round focused on communication and my ability to explain complex technical topics. They were interested in understanding how well I could explain the work I did in previous projects and how updated I was with the latest technology and trends.
Q: How did they check whether you worked on your projects or just used someone else’s work?
They asked specific questions about the projects, such as how I approached the problem and the challenges I faced. This helped them assess whether I had truly done the work or if I was using proxies.
Q: What happened in the technical rounds?
The fourth and fifth rounds were both technical rounds. They were deep dives into the technical aspects of the projects I had worked on. I had to explain the models I built, the algorithms I used, and the reasoning behind my decisions.
Q: How was the sixth round different from the others?
The sixth round was more about overall evaluations. I had discussions with senior people, including directors and vice presidents. They asked me about my approach to problem-solving, my communication skills, and how I would handle different types of projects in real-world scenarios.
Q: Were these final rounds focused purely on technical skills, or was there a mix of skills assessed?
It was a mix. While they focused on technical knowledge, they also evaluated how well I could communicate those skills, how I worked in a team, and whether I could fit into their organizational culture.
Q: How were these final discussions structured?
The discussions revolved around real-world applications of the concepts I worked on. They wanted to know how I would handle complex situations, communicate with stakeholders, and solve problems creatively.
Q: What advice would you give to others preparing for Data Science interviews?
My advice is to practice, practice, and practice more. You need to understand your code and the approach you are using, rather than simply copying solutions from the internet. Even small errors can take up a lot of time to troubleshoot, so gaining a deep understanding of your work is key.
Q: How important is it to understand the error and debugging process in Data Science?
Debugging and understanding errors are crucial. Sometimes, issues arise due to small things like version mismatches in libraries, and it can take a lot of time to pinpoint the exact problem. So, it's vital to have a solid understanding of both the theoretical and practical aspects of your projects.
Q: Can you elaborate on what specific skills candidates should develop for success in the field of Data Science?
Apart from technical skills, I’d recommend building your problem-solving abilities and creativity. There are often multiple ways to approach a problem in Data Science, and being able to think creatively can set you apart from other candidates.
Q: How do you recommend building a portfolio of Data Science projects?
Start by selecting interesting problems, work on them from scratch, and document your journey. Make sure you understand every part of the project, from data cleaning to model deployment. This will give you a portfolio that shows your capability.
Q: There’s a concern about AI taking over jobs. How do you think Data Science professionals will fare in the future with this trend?
It’s true that AI is automating a lot of jobs, but Data Science professionals are safe for the time being. The demand for skilled Data Scientists is only going up, as AI still requires human intelligence for problem-solving, model tuning, and real-world applications. The growth in AI and machine learning has also led to an increase in job opportunities, especially in tech companies.
Q: Do you think the growth of AI will impact job availability for Data Scientists?
While AI is automating some tasks, it’s creating more opportunities for Data Scientists. The need for human oversight, creativity, and domain-specific knowledge in solving real-world problems ensures that Data Science will remain a growing field for years to come.
Q: What are your thoughts on people entering Data Science with non-technical backgrounds, such as Finance?
It’s not as difficult as it might seem. If you have a strong understanding of mathematics and statistics (as you likely would in finance), learning the technical aspects of Data Science can be manageable. Once you grasp Python and the theory behind machine learning models, you’ll be on your way.
Q: Do you need to know Python to be a Data Scientist?
Yes, Python is essential. It’s the primary language used in the field and is relatively easy to learn. I recommend starting with basic Python programming and then diving into libraries like Pandas, NumPy, and Scikit-learn. Once you get the hang of it, you can start experimenting with more advanced topics like deep learning and NLP.
Q: As a final piece of advice, what would you suggest for someone starting out in Data Science?
My final advice would be to stay curious and never stop learning. Practice regularly, work on real-world projects, and most importantly, understand the theory behind the algorithms and techniques you're using. Don’t just focus on coding; focus on how and why things work the way they do.
Refer these articles:
- Becoming a Data Scientist: Atif Ansari’s Inspiring Career Leap
- From Civil Engineering to Data Science: Prasad’s Inspiring Journey
- Komal’s Path to Data Science: A Journey of Growth and Success
Insights from Dinesh's Path to Data Science Mastery
Dinesh's journey to mastering data science is a testament to perseverance, continuous learning, and the ability to adapt to rapidly changing technologies and methodologies.
- Dinesh transitioned from a mechanical engineering background with no prior technical experience in IT.
- He worked for 1.5 years as a supervisor in inventory management before switching to data science.
- He enrolled in a Data Science course at DataMites around September to kickstart his career in the field.
- A mechanical background encourages problem-solving and innovation, which aligns well with data science requirements.
- Emphasis on practical, hands-on projects rather than just learning theory or copying code.
- Recruiters focus on creative approaches and a strong understanding of projects during interviews.
- He went through a 6-round interview process, including aptitude, technical, and managerial rounds.
- Debugging skills and practice are vital — minor errors and version issues can cause major delays.
- Communication and stakeholder explanation skills were tested in specific interview rounds.
- The final interview rounds included senior leadership, such as directors and VPs, indicating role importance.
- Recruiters verify project authenticity to ensure candidates didn’t submit copied or externally done work.
- Aptitude tests were rated as easy to moderate, manageable with basic preparation.
- Companies often give take-home project assignments with short deadlines (1–2 days).
- He noted that finance and other non-technical professionals can also transition into data science.
- Staying updated on current AI/ML trends is important, as interviews often test domain awareness.
Refer these articles:
- Why Data Scientist Career in Bangalore
- Why Data Scientist Career in Kolkata
- Why Data Scientist Career in Mumbai
The data science interview process is evolving and becoming more holistic. Companies are now focusing on not just technical skills but also communication, project ownership, and adaptability. The candidate’s journey with IQ Gateway proves that with genuine curiosity, strategic preparation, and a commitment to learning, anyone can crack even a six-round interview process.
If you're contemplating a transition into data science, there's no better time than now to upskill. As per Precedence Research, the global data science platform market was valued at USD 150.73 billion in 2024, is projected to grow to USD 175.15 billion in 2025, and is expected to exceed USD 676.51 billion by 2034 — reflecting a strong CAGR of 16.20% from 2024 to 2034. This remarkable expansion is fueled by the rapid increase in digital data generation across various industries. To remain competitive in this evolving landscape, choosing a training institute that focuses on hands-on experience, real-time internships, and strong placement assistance is essential. Enrolling in a reputed data science course in Mumbai, Pune, Bangalore, Hyderabad, Chennai, Kolkata, and Delhi can be the first step toward building a strong tech career.
DataMites Institute has positioned itself as a leading provider of training in Data Science, AI, Machine Learning, Python Development, and Data Analytics. Backed by IABAC and NASSCOM FutureSkills accreditation, DataMites delivers expert-led instruction, real-time project work, and placement support that enables smooth career transitions into the world of data science.
With offline Data Science training in Bangalore, Hyderabad, Chennai, Pune, Ahmedabad, Coimbatore, and Mumbai, along with flexible online learning options, DataMites offers accessibility and flexibility for learners across backgrounds. Its practical, industry-focused curriculum equips freshers, working professionals, and career switchers with the skills needed to thrive in data-driven roles.
This story is a testimony that success doesn't come only from expertise but from resilience and continuous improvement. Dinesh’s journey from a mechanical engineer to a data scientist highlights how determination, the right guidance, and hands-on experience can lead to a successful career switch. His story proves that with dedication, even those from non-tech backgrounds can thrive in the field of data science.