Becoming a Data Scientist: Atif Ansari’s Inspiring Career Leap
Atif Ansari’s journey to becoming a Data Scientist showcases a remarkable career transformation fueled by dedication and upskilling. His story highlights how the right training and perseverance can lead to success in the data science field.
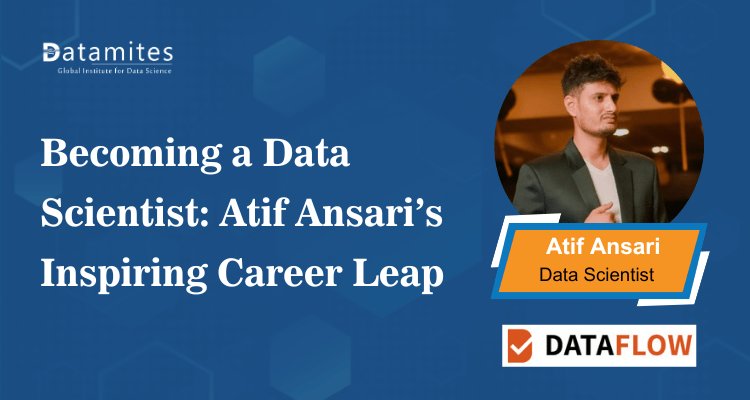
Career transitions are never easy—especially when moving from a non-technical domain to one rooted deeply in programming and analytics. But Atif's journey from mechanical engineering to becoming an Associate Data Scientist proves that with the right mindset, training, and perseverance, it’s entirely achievable.
In an engaging conversation with DataMites, Atif opens up about his transition journey, challenges, learning curve, and eventual success. Here's a comprehensive walkthrough of the insights he shared, presented in a Q&A format for clarity and impact.
Atif Ansari's Journey into Data Science: A Story of Transformation with DataMites
From facing career uncertainty to becoming a successful data scientist, Atif Ansari's journey with DataMites is a testament to perseverance and focused learning.
Q1: Can you briefly introduce yourself?
I’m Atif Ansari. I hold a degree in Mechanical Engineering and worked in that domain for over four years. However, I didn’t see the growth I aspired for, so I decided to transition into data science and AI after extensive research. I enrolled in the DataMites institutes six-month program around December 2021 to make that switch smoother.
Q2: How did you approach this career change, considering your background?
Since I had no background in programming, I knew it would be tough. I went with DataMites because they offered a clear learning path and good support. Their course, hands-on projects, and internship gave me solid preparation for working in the industry.
Q3: Where are you working currently and in what role?
I’m working as an Associate Data Scientist at DataFlow Group in Noida. I’ve been with the company for over a year now.
Q4: How was programming for you at the beginning?
Honestly, I didn’t even know how to write a basic “Hello World” program when I started. It was challenging, but I focused on developing analytical thinking and logical reasoning, which are just as important as coding in data science.
Q5: What kinds of projects have you worked on at your current job?
I’ve worked on multiple NLP-based projects. One involved extracting details from resumes for HR operations. Another key project focuses on detecting document forgery—identifying tampering or overwriting using OCR and NLP techniques.
Q6: Did you pursue the AI Expert program as well?
No, I only completed the Certified Data Scientist (CDS) program. The rest of my learning—especially on LLMs and advanced NLP—has been self-driven.
Q7: Was statistics a new subject for you?
Mostly, yes. In mechanical, I dealt with basic analytics while planning machine jobs, but I wasn’t deeply involved with statistics. Through DataMites, I learned applicable statistical concepts that I still use in the industry.
Q8: How relevant was the statistics portion of your course to real-world work?
Atif: Extremely relevant. The syllabus was focused and practical. It wasn’t about reading thousands of pages but rather learning what’s actually useful in industry scenarios.
Q9: What tools and skills are essential for aspiring data scientists today?
Beyond programming, you should understand NLP, computer vision, SQL, and BI tools. Strong logical thinking and conceptual knowledge are crucial, especially when solving business problems during interviews.
Q10: How often do you use SQL at work?
Almost daily. SQL is essential for working with databases—it helps you access, link, and change data. It’s a fundamental skill for any data-related job.
Q11: How valuable was the PAT (Placement Assistance Team) mock interview experience?
Very valuable.I didn’t do well in my first mock exam, and my performance in the second one wasn’t much better. But I took the feedback constructively, worked on it, and improved significantly. It really helped me prepare for real interviews.
Q12: What was your study pattern like?
My routine wasn’t consistent. Some days I didn’t study at all, and other days I’d study for 6–8 hours. But I never missed a class and always made detailed notes, which I still refer to before interviews.
Q13: Is daily study necessary to succeed in this field?
Not necessarily. Even studying two to three hours daily is enough if you’re consistent. Attending every class and creating thorough notes were the game changers for me.
Q14: How important is conceptual thinking in data science?
Extremely important. It helps in solving real-world problems. Programming can be outsourced or improved later, but core analytical thinking must be developed personally.
Q15: Have you worked on any white papers or research projects?
Yes. I recently completed a research paper on generative AI. It explores models that allow online teachers to gauge student engagement and understanding during virtual classes—mimicking the classroom feedback loop.
Q16: Is publishing a paper beneficial for job seekers in this field?
Absolutely. It gives credibility to your expertise and adds serious weight to your profile during interviews. White papers are an effective way to demonstrate strong knowledge of a subject.
Q17: How valuable is an internship after completing the course?
Invaluable. Internships give you a chance to implement what you've learned. During interviews, being able to speak about real-world application of your knowledge gives you a solid edge.
Q18: What advice would you give someone from a non-technical background who wants to transition into data science?
It’s definitely possible. Your previous domain knowledge is still useful. Focus on building your programming, statistics, and problem-solving skills. Internships and projects will give you hands-on experience.
Q19: What kind of projects should learners focus on?
Projects that reflect real-world problems—like resume parsing, document verification, or sentiment analysis. Try covering all major areas: NLP, CV, ML, and basic AI. Employers love practical experience.
Q20: What are your future plans in data science?
I’m passionate about NLP. I plan to stay focused in that domain and continue working on projects and learning more about large language models (LLMs). Even in my personal time, I work on NLP-related tasks.
Q21: What should I do if I get stuck or fail in an interview?
Reflect on where you got stuck, identify the gaps in your knowledge, and work on them. Every interview is a learning opportunity. Use failures to guide your preparation.
Q22: Is not getting selected in an interview a sign of failure?
No, not at all. Many factors affect the outcome of an interview — the interviewer’s mindset, expectations, and what they’re looking for at the moment. Not being selected doesn't mean you're not good enough.
Q23: How important is consistency in interview preparation?
Very important. Keep trying and don’t quit. Consistent learning and improvement are key to succeeding in the long run.
Q24: What should candidates focus on when preparing for interviews?
Focus on improving yourself continuously. Work on the areas where you struggle, and make sure you understand the fundamentals. Also, take note of feedback and learn from each experience.
Q25: Does success in interviews depend only on preparation?
No, preparation is crucial, but success also depends on whether your skills and approach match what the company is looking for. Sometimes, even well-prepared candidates may not get selected due to misalignment.
Q26: Any advice for those currently transitioning into new roles or careers?
Keep learning, stay motivated, and don’t see rejections as failures. Stay persistent and focus on continuous improvement. Eventually, the right opportunity will align with your efforts.
Refer these articles:
- From Civil Engineering to Data Science: Prasad’s Inspiring Journey
- Komal’s Path to Data Science: A Journey of Growth and Success
- From Beginner to Expert: Sunil Kumar's Data Science Journey
Insights from Atif Ansari’s Journey to Success in the Data Science Field
His story highlights the power of perseverance, upskilling, and the right guidance in achieving a successful career transition into data science.
Career Transition from Mechanical to Data Science
Atif moved from a mechanical engineering position, where he had over 4 years of experience, to a career in data science because he saw limited opportunities for advancement in his previous field.
Strategic Decision & Course Selection
After thorough research, he chose DataMites to gain structured guidance in data science and AI.
Learning Curve with No Programming Background
Despite starting with no coding knowledge, he built programming skills gradually through consistent effort.
Conceptual Thinking Over Pure Coding
Emphasizes that logical reasoning, analytical ability, and conceptual clarity are as important as coding in data science.
Completion of Course + Internship
Finished the course (Dec 2021–mid-2022) along with capstone projects and a hands-on internship that bridged theory and real-world practice.
First Data Science Job at DataFlow Group
Landed a role as Associate Data Scientist, applying AI in OCR, NLP, and document verification projects.
Tools & Technologies in Daily Use
Uses SQL, Python, and Power BI regularly—skills gained directly from the DataMites curriculum.
Overcoming Interview Challenges
Participated in mock interviews, embraced feedback, and used interview setbacks as opportunities to grow.
Research and Advanced Learning
Authored a white paper on Generative AI and pursued self-learning in NLP and Large Language Models beyond the curriculum.
Study Approach: Consistency with Intensity
Maintained detailed notes, attended all classes, and studied intensely rather than daily, showing disciplined learning habits.
Rejections Are Not Failures
Believes interview rejections should be seen as learning opportunities, not as signs of inadequacy.
Persistence and Self-Belief
Highlights the importance of being steady and having a positive attitude while looking for a job and learning new things.
Inclusivity in Data Science Careers
Encourages people from non-tech backgrounds (like Pharma or Mechanical) to enter data science with determination and effort.
Future Aspirations in NLP and LLMs
Plans to deepen expertise in NLP and generative AI, reflecting a clear direction for career growth.
Refer these articles:
- Why Data Scientist Career in Chennai
- Why Data Scientist Career in Bangalore
- Why Data Scientist Career in Kolkata
Atif’s journey from mechanical engineering to data science wasn’t linear or easy. But his story is a testament to the power of structured learning, perseverance, and strategic effort. For aspiring data scientists, especially those coming from non-coding backgrounds, Atif’s experiences offer both inspiration and a roadmap. According to Precedence Research, the global data science platform market is projected to grow from USD 150.73 billion in 2024 to approximately USD 676.51 billion by 2034, at a CAGR of 16.20%.
For aspiring professionals like Atif, enrolling in offline data science courses in Chennai, Hyderabad, Bangalore, Pune, Mumbai, Delhi, and Kolkata can provide a solid foundation and a competitive edge in this high-growth sector.
To remain ahead in this fast-evolving field, it's essential to choose a training provider that emphasizes hands-on experience, internships, and strong placement support.
DataMites Institute is one such leading training provider, renowned for its expertise in Data Science, Artificial Intelligence, Machine Learning, Python Programming, and Data Analytics. Accredited by IABAC and NASSCOM FutureSkills, DataMites offers industry-relevant, high-quality training delivered by experienced instructors.
DataMites emphasizes hands-on learning through real-time projects and internship experiences, coupled with comprehensive placement assistance, ensuring that learners like Atif acquire both the necessary skills and industry exposure for career growth. Additionally, DataMites offers offline data science training in Bangalore, Hyderabad, Chennai, Pune, Ahmedabad, Coimbatore, and Mumbai.
No matter your current level, you can begin learning programming, statistics, NLP, or SQL from there. With the right resources like DataMites and a commitment to continuous learning, your transition into a high-growth field like data science is more than just possible—it’s within reach.