Data Science Challenges and How to Overcome Them
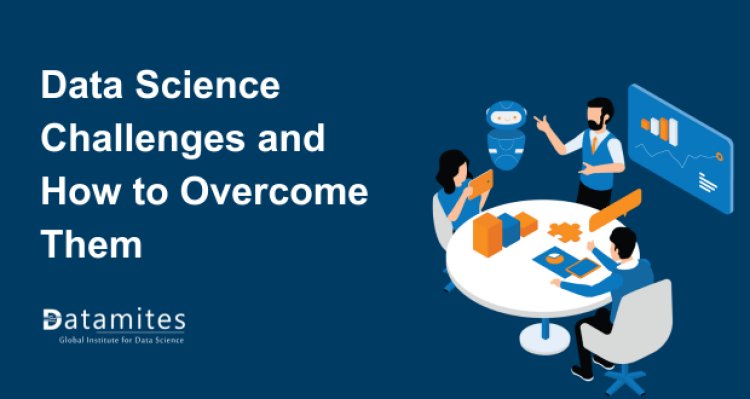
In today’s digital age, data science is transforming industries by facilitating data-driven decisions that enhance efficiency, boost customer satisfaction, and increase profitability. With the growing recognition of data’s value, the demand for skilled data scientists has surged dramatically; reports indicate that the data science job market is expected to grow by 22% over the next decade, significantly outpacing the average for other occupations. Additionally, a recent analysis by the World Economic Forum predicts that by 2030, there will be 700,000 new job openings in this field, highlighting its vast potential and scope.
However, despite the promising career opportunities, data science presents several challenges. Recognizing and effectively addressing these obstacles is crucial for success in this fast-paced and continually evolving field. This blog will delve into the various challenges faced by data scientists and provide practical solutions to tackle them. If you are considering a career in data science, you will discover valuable strategies and tips to enhance your learning experience and become a skilled data scientist.
Understanding Data Science: The Backbone of Modern Innovation
Data science involves examining extensive datasets to identify patterns, derive insights, and forecast future trends in Data Science.It integrates disciplines like statistics, computer science, and domain-specific knowledge. Data scientists utilize techniques like machine learning, data mining, and predictive analytics to assist organizations in making strategic and informed decisions.
The career potential in data science is massive, driven by the demand across multiple sectors, including healthcare, finance, and technology. The growth of big data, artificial intelligence, and automation has only accelerated this trend. Enrolling in a Top Data Science course or a Data Science certification course from a reputable Top Data Science institute can be a great way to tap into these career opportunities. These programs provide not only foundational knowledge but also hands-on experience with real-world data sets.
Refer these articles:
- Top Data Science Certifications to Boost Your Career
- Data Science Project Lifecycle
- Data Science Applications
Common Challenges in the Field of Data Science
While data science holds great promise, it also comes with numerous obstacles that professionals must overcome to succeed. Let’s take a closer look at some of these challenges.
1. Poor Data Quality
One of the most fundamental challenges in data science is poor data quality. Data can often be incomplete, inconsistent, or outdated, leading to flawed analysis and unreliable insights. When data lacks quality, the models built on it are less effective, reducing their usefulness for decision-making.
To mitigate this, data scientists must spend a significant amount of time cleaning and preparing data. They need to identify missing values, remove duplicates, and ensure data accuracy before any analysis begins.
2. Data Silos
Many organizations store data across different systems, making it difficult to access and analyze as a whole. This problem, known as data silos, limits the ability to gain a comprehensive view of business performance and customer behavior.
To address this, companies need to prioritize the integration of their data. Breaking down these silos by centralizing data storage or creating systems that allow for easy data sharing can greatly enhance the effectiveness of analytics efforts.
3. Shortage of Skilled Professionals
While the demand for data scientists is growing, there is a significant gap in the availability of skilled professionals. Many organizations struggle to find experts who possess both technical know-how and business acumen. This shortage makes it harder for companies to keep up with their data science initiatives.
One way to overcome this challenge is by investing in Data Science training. Organizations can encourage their employees to upskill by enrolling in a Data Science certification course, which provides specialized training in areas such as machine learning, data visualization, and big data technologies.
4. Complex Data Integration
Data comes from various sources, such as social media, databases, sensors, and cloud services. Combining data from these diverse sources into a unified format is a complex task. Each data source has its structure, and harmonizing them is often a time-consuming process.
Data integration tools and platforms, such as ETL (Extract, Transform, Load) pipelines, can help streamline this process. By automating data extraction and ensuring consistency during transformation, these tools make it easier to analyze integrated data effectively.
5. Data Privacy and Security Concerns
Data security is a top priority for businesses, especially when dealing with sensitive or personal information. Any breach in data security can result in legal complications and loss of customer trust. With growing regulations like GDPR and CCPA, ensuring data privacy has become even more crucial for companies working with large data sets.
Organizations need to invest in strong encryption methods, secure storage systems, and regular security audits. Building a data governance framework that includes privacy and security policies is also essential for maintaining compliance with regulations.
6. Communicating Insights Effectively
Communicating data insights in a way that is easily understood by non-technical stakeholders can be challenging. Data scientists often struggle to translate complex findings into actionable recommendations for teams without a technical background.
To bridge this gap, data scientists must improve their storytelling and data visualization skills. Using clear visuals and focusing on key takeaways can help stakeholders grasp the importance of data insights and make more informed decisions.
7. Keeping Up with Technological Changes
Data science is a fast-changing domain, constantly seeing the introduction of new tools and methodologies. Staying updated with the latest developments can be overwhelming, but it’s crucial for maintaining a competitive edge in the field.
Continuous learning is the best way to keep pace with these changes. Professionals should regularly attend industry conferences, enroll in advanced Data Science training, and subscribe to online courses to stay informed. Keeping your Data Science skills sharp will not only enhance your career prospects but also improve your ability to navigate future challenges.
8. Managing Expectations
Organizations frequently have elevated expectations regarding the outcomes that data science can achieve. However, data science projects can be complex and time-consuming, and stakeholders may expect immediate results. It is essential to manage these expectations to prevent unrealistic demands.
Setting clear goals and timelines at the beginning of a project helps ensure everyone is on the same page. Regular updates and communication with stakeholders will also help manage their expectations and prevent potential misunderstandings.
Refer these articles:
Strategies to Overcome Data Science Challenges
Overcoming challenges in data science is essential for successful project execution and achieving meaningful insights. Here are several strategies to address common data science challenges:
1. Data Quality Management
Ensuring high data quality is crucial, as poor data can lead to inaccurate insights. Implementing robust data governance practices, such as validation checks and outlier detection, can help maintain data integrity. Regular cleaning and automated monitoring tools enhance data quality throughout its lifecycle.
2. Skill Gap and Talent Shortage
The fast-paced evolution of data science creates a skill gap. Organizations can address this by investing in training and development programs, offering workshops, online courses, and certifications. Collaborating with academic institutions can also help build a skilled talent pipeline.
3. Data Silos and Integration Issues
Data silos hinder comprehensive analysis. Promoting a centralized data strategy and implementing data warehouses or lakes can facilitate integration. Adopting open data standards and fostering cross-department collaboration can also dismantle silos and enhance data utilization.
4. Managing Expectations and Communication
Unrealistic stakeholder expectations can lead to dissatisfaction. Clear communication is essential for managing these expectations. Data scientists should provide regular updates, articulate complexities, and use data visualization tools to present findings in an accessible way.
5. Algorithm Selection and Model Overfitting
Choosing the right algorithm and avoiding overfitting are critical in model development. Employing techniques like cross-validation and regularization can help. Using a variety of algorithms and ensemble methods enhances model robustness and performance.
6. Ethics and Bias in Data Science
Addressing bias and ethical considerations is vital. Data scientists should implement fairness metrics and bias detection tools. Engaging a diverse team can highlight potential biases, and establishing ethical guidelines fosters accountability in data usage.
7. Deployment and Maintenance Challenges
The transition from model development to deployment presents challenges like integration and scalability. Adopting MLOps practices facilitates collaboration between data scientists and IT teams, ensuring smooth deployment and ongoing model maintenance through monitoring and clear documentation.
In conclusion, addressing data science challenges demands a multifaceted approach that encompasses technical solutions, organizational strategies, continuous learning, and strong ethical practices. By applying these strategies, organizations can not only enhance their data science capabilities but also unlock valuable insights from their data. For those pursuing a career in data science, overcoming these challenges is essential for staying competitive and advancing in this rapidly growing field, enabling them to contribute meaningfully to the success of their organizations.
Refer these articles:
- Data Science Course Fee in Bangalore
- Data Science Course Fee in Chennai
- Data Science Course Fee in Pune
Importance of Overcoming Challenges
Overcoming challenges in data science is essential for both professionals and organizations to fully realize the benefits of this field. The importance lies in several key areas:
- Maximizing the Value of Data: Data science is all about deriving insights from vast amounts of data. Challenges such as data quality, privacy concerns, or the complexity of large datasets can hinder this process. By overcoming these obstacles, data scientists can extract accurate, actionable insights that drive better decision-making and innovation.
- Staying Competitive in the Job Market: With the rapid growth in demand for data scientists, the field is becoming increasingly competitive. Those who can navigate challenges like mastering complex algorithms, handling unstructured data, and keeping up with emerging tools and technologies will stand out in the job market. Overcoming these challenges allows professionals to continually enhance their skills, making them valuable assets to employers.
- Improving Organizational Outcomes: For companies, overcoming data science challenges directly impacts business outcomes. Successfully addressing issues such as integrating data from multiple sources or implementing advanced analytics solutions leads to greater efficiency, cost savings, and improved customer experiences. This is critical for organizations aiming to stay ahead in a data-driven economy.
- Driving Continuous Innovation: Data science is a constantly evolving field with new technologies and methodologies emerging regularly. Overcoming challenges such as adapting to new tools or improving machine learning models fosters innovation. It enables professionals and businesses to stay on the cutting edge, leading to breakthroughs and long-term success.
In summary, overcoming the inherent challenges in data science is crucial for achieving personal growth, maintaining a competitive edge, and driving both organizational and industry-wide innovation.
For those aspiring to enhance their skills, DataMites Institute presents a variety of advanced training programs specifically designed to align with the ever-evolving requirements of the data-driven industry. As a premier provider of education in data science and artificial intelligence, DataMites partners with respected organizations such as the IABAC and NASSCOM FutureSkills. These all-encompassing programs empower professionals with cutting-edge skills and critical industry insights.
In addition, DataMites offers a wealth of resources to support learners, including workshops, webinars, and job placement assistance, all aimed at facilitating professional development. Whether you're embarking on your data science journey or looking to elevate your career, DataMites equips you with the necessary tools and mentorship to thrive in this dynamic field. Step into the future with DataMites and unleash your potential in the exciting world of data science!