How the Financial Sector is Leveraging Data Science
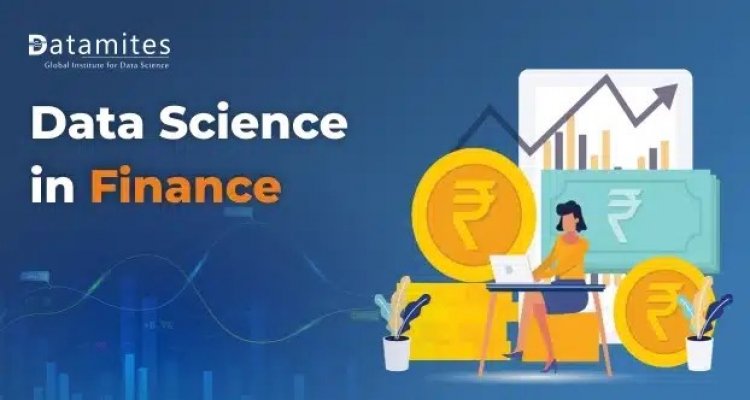
Without a doubt, big data has revolutionized our economy. Possibly the finest illustration of this is the impact it has had on the global finance industry. Finance has benefited greatly from the digital revolution as one of the first businesses to completely embrace big data.
Putting Data Science into Practice
Data science has started to be incorporated into domains that previously showed little interest in its development. On the other hand, the finance sector has long understood the benefits of data science. Since then, data science applications in finance have grown quickly, becoming integral to the sector’s operations.
Today, the banking and financial sectors heavily rely on data science and it is possible to say that data science and finance actually complement each other. The services they presently provide range from personalized online banking to automated pricing! Indeed a long leap! Let’s take a look at the top data science applications in the field of finance.
- Boost in Sales and Income
Numerous financial institutions feel the need to improve their client involvement through valuable personal relationships. Data Science gives businesses the ability to monitor whether or not their customers are using better amenities. It assists in opening up a totally new level of individualized consumer experience.
Data science in finance can examine consumer behavior and provide insightful data that helps various businesses provide their customers with the best services in real time. Thereby the businesses benefit from being better able to predict future client behavior and value generation.
- Keep Risks at Bay
Risks are an inherent aspect of operating a business. Risks should be assessed, and conclusions should be drawn so that the proper precautions will be taken the next time.
Risk analytics is one of the most useful applications of business intelligence and data science in finance. Risks associated with operating a business must be recognized, assessed, and controlled as part of the risk management process.
Fraud in financial institutions is a serious problem. The volume of transactions in the country has skyrocketed, and so have instances of fraud and cybercrime. Effective risk management is the sole method of increasing the financial institution’s profitability. Data science in the financial markets has made risk management much easier nowadays. With the use of risk analytics and management, a firm may make strategic decisions, enhance its credibility, and increase its security. For minimizing risks, data science is the winner.
- Getting Valuable Information
This is directly related to the previous point, or we could say that it results from keeping the risks on tabs. The study of fraud has produced data-driven insights, such as the existence of dependable clients with a track record of purchases, which can benefit the company and lessen the risk of fraud.
However, financial activities and parameters are constantly monitored when riskier clients are involved. This aids financial firms in determining the best course of action for the next phase of their operations while keeping in mind all the information they have gathered.
- Insights on Consumers
Understanding consumer behavior is essential for any bank or provider of financial services to use as a guide when making choices. And the finest technique to comprehend customers is through their data.
Every financial institution strives to bring personalization to its consumers. Companies can use real-time analytics and data scientists to obtain insights into consumer behavior in order to make better decisions. Banks, insurance companies, pension funds, and credit card businesses can obtain very specific insights by combining several data sources and employing demographics like age and geography. These insights allow them to adjust their direct marketing and client relationship management strategies as necessary. This could be utilizing data to upsell specific products or enhance customer service.
Refer this article: Support Vector Machine Algorithm (SVM) – Understanding Kernel Trick
- Individualized Services
Prior to this digital revolution, banking processes were literally physical. But, with the online platforms coming into emergence, transactions became extensively online. Personalized services are a terrific strategy for a business looking to keep up strong relations with its clients and boost sales. In order to market the services they have to give, financial data scientists from various institutions determine the customer’s interests.
The task of financial data scientists is made easier by the tools for language processing and speech recognition. Customers’ requirements are recognized, and the firm gains useful insights that may be put to use. These insights are also applied to improving and personalizing consumer services. Indeed, there are a plethora of tailored options available while making transactions online, the financial sectors have come a long way.
What is Covariance
- Computerized Trading
Big data has significantly changed computers and the way new financial strategies are being developed for commercial companies. Massive amounts of data are converted into simplified information via algorithmic trading. As a result, financial institutions can effectively forecast the financial markets.
Algorithmic trading is used to quickly develop new financial strategies with the aid of large data. The procedure is now entirely automated, allowing for extremely quick purchasing and selling. In fact, the algorithms used are so astonishingly quick that they have inspired a new market practice. However, the scene has changed as a result of the introduction of big data and real-time insights. The speed of trade eventually surpassed what humans could handle.
- Product Development
FinTech, most commonly known as Financial Technology is the industry that is experiencing quicker growth rates at the moment. Although this young sector has only just evolved, it has been fast to capitalize when compared to the traditional banking systems and how they worked. Nowadays, FinTech startups are delivering interesting breakthroughs at a rate faster than what multinational corporations can handle since they are making the entrance into the market with a disruptive start-up mindset.
Digital banks have been introduced by several fintech companies, although others concentrate on particular technological fields before selling these. A few examples of fintech that is fueled by data science include blockchain and cryptocurrencies, mobile payment systems, trading apps that are analytics-driven, lending software, and insurance solutions that use AI.
- Managing Customer Data
Data is everything, and in order to handle and analyze the information, the financial institution requires customer data. Despite the fact that most of the data nowadays is digital, the bulk is completely unstructured. Adding order to this mess is difficult because real-time data is continuously coming in.
After big data was introduced to the field of data science, the way financial institutions operate has undergone a complete upheaval. Customer purchases and purchasing patterns are tracked by customer data management companies. Their preferences and decision are considered. Data science in finance assists in studying client purchase patterns and providing customers with the services they like.
- Allocation of credit
Funds are given to the customer through a variety of financial services. Underwriters and credit ratings, which are determined by credit rating organizations based on an applicant’s prior repayment history, are traditionally used to evaluate an applicant’s creditworthiness.
The process of allocating credit may be made considerably more informed with the help of data science, though. Predictive models that assess the creditworthiness of the customer by considering not only the credit score but also the demographic information and other digital footprints like social media, reviews, or anything related to customers can help underwriters make wise decisions.
Read this article: 10 Python Built-in Functions Which You Should Know While Learning Data Science
- Decision-making
Probabilistic or statistical The future performance of the assets is frequently predicted through analysis. This assists financial institutions in important decision-making and lowers the possibility of errors. But because the world is constantly changing, building models that not only function effectively but also quickly adapt when given new information has become crucial.
Certain decision-making issues required more than statistical analysis, such as choosing when to buy stocks, where models based on machine learning and artificial intelligence are the only solutions. For example, machine learning can assist in stock market forecasting.
What is Boosting
Data Science in Finance – The Future
It cannot be disputed that the adoption of data-driven approaches, data science tools, and algorithms has greatly aided the expansion of the financial sector. Using client data that is already available, data science in finance can see trends and produce findings that are more complete. Strong tactics and decisions are made possible by putting cutting-edge data science techniques into practice.
Many big data firms create predictive algorithms that can comprehend and modify data sets, and process enormous amounts of informed decisions. Data science does help produce a more in-depth comprehension of patterns and makes institutes stand further ahead of the competition. Indeed better financial solutions are predicted for the future, with data science ruling the finance industry and making it easier for financial institutions to move ahead and flourish.
As vital and pertinent as it is in other commercial design-making fields, data science is equally important in the finance sector, most specifically banking. The data science experts in the finance industry provide support and assistance to the appropriate teams inside the organization, particularly the investment and financial teams. Not a wonder, given how profound the applications of data science are in the present day. Due to the increasing use of data science in organizational and decision-making processes by industries worldwide, data science professionals are in great demand.
Also refer this article: Data Science Vs Data Analytics
Final say
Data science has expanded tremendously in recent years, and so have its uses across many different industry sectors. Data science and finance are closely related because finance is the hub of data. Financial institutions were among the pioneers in data science and analytics adoption and innovation, and they do fully stand to reap the benefits of it.
You’ve come to the correct place if data science fascinates you. DataMites is a leading global source of data science training, and its curriculum was carefully designed to address the expanding needs of the sector. The flagship course we provide, the DataMites Certified Data Scientist Course, is eight months long and will teach you everything you need to know about data science, from level zero to level ten. Our data science courses meet the highest standards thanks to their accreditation from NASSCOM, Jain University, and IABAC.
Bias-Variance – Data Science Terminologies