Inspiring Data Science Journey of Yash Sahindrakar
Yash Sahindrakar's data science journey showcases his transition from a curious learner to a skilled professional through relentless self-learning and hands-on projects. His story inspires aspiring data scientists to stay committed and embrace continuous growth.
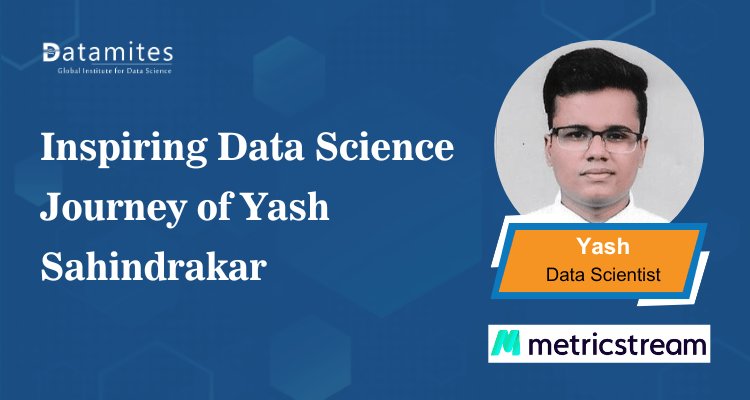
In an age where technological advancement has revolutionized the job landscape, many professionals are pivoting toward the IT sector—and for good reason. One such inspiring journey is that of Yash, a mechanical engineering graduate who successfully transitioned into a data scientist role, thanks in part to his time at DataMites.
We will explore Yash’s story from skepticism to success, how he navigated his career shift, and the insights he shares for aspiring data professionals. If you're someone looking to break into data science or data analytics, Yash's experience will serve as both a roadmap and a motivation booster.
Yash’s Path to a Successful Data Science Career with DataMites
A remarkable story of how dedication, the right mentorship, and upskilling turned a mechanical engineer into a successful data scientist.
Q1. Could you share a little about your background and what motivated you to join DataMites?
I graduated in mechanical engineering, but I was skeptical about switching careers to IT. The mechanical job market wasn’t great at that time, so I decided to look for courses in IT. That’s when I found DataMites Institute data science course and immediately enrolled.
Q2. How was your experience at DataMites?
After joining DataMites, my outlook shifted entirely—the course ignited my curiosity to explore new areas like Python and machine learning, which I was initially doubtful about. The outstanding guidance and encouraging instructors enabled me to conquer my doubts, boost my confidence, and understand challenging concepts with ease.
Q3. Did you have any prior programming knowledge before joining?
None at all. I started from absolute scratch, but because of the structured approach at DataMites, I didn't find it difficult to catch up.
Q4. What was your learning strategy during the course?
I focused first on building a solid foundation. I began with basic Python, SQL, and even Excel, before moving on to machine learning. I didn’t jump straight into advanced topics like AI; I made sure my base was strong first.
Q5. How did your first job opportunity come about?
Within just 3–4 months of starting the course, I landed my first job as a Software Engineer at a startup, CEST Aerospace. The Python skills I gained from DataMites played a significant role in cracking the interview.
Q6. What was your path after your first role?
I transitioned into a Power BI Consultant/Data Analyst role, where I worked for around 1.5 years. After that, I secured a Data Scientist role in a product-based company, working on cutting-edge technologies including LLMs and cybersecurity.
Q7. What kind of questions were asked during your data science interviews?
The interview primarily revolved around my dissertation work from my Masters at Liverpool John Moores University. They focused on deep learning, computer vision, and also tested my Python skills.
Q8. Did any project from DataMites help during interviews?
Yes!I presented a deep learning project focused on detecting leaves. Interviewers asked about the choice of architecture, such as CNN vs Transfer Learning, and it gave me an edge.
Q9. Which resources did you use to prepare for interviews?
I practiced Python on HackerRank, followed LinkedIn communities, and read industry-relevant articles. These helped me stay updated on current trends like GenAI and LLMs.
Q10. What does a day in your life look like as a data scientist?
It involves R&D, optimizing code, building and testing ML architectures, and exploring tools like AWS Lambda. It’s not just coding—I also work on some UI and cybersecurity tasks depending on project needs.
Q11. Which tools and platforms do you use regularly?
I extensively use AWS services, especially Lambda functions, which rely on Python. In past roles, I’ve also used Power BI and a proprietary tool called SM Report B for analytics.
Q12. For someone not keen on coding, is there an alternate path?
Absolutely. Roles like Data Analyst or Business Analyst are more low-code and focus more on reporting, visualization, and data interpretation. These can be great entry points.
Q13. Besides Python, what are other essential skills for data scientists?
Along with Python, Machine Learning, SQL, and domain knowledge (like NLP or Computer Vision) are crucial. You need to specialize in at least one of these areas for advanced roles.
Q14. How did you approach your job search?
LinkedIn was my go-to platform. I optimized my resume for each job role—highlighting relevant domain-specific projects. My current job came via referral, again thanks to networking.
Q15. How important is a well-crafted resume?
Extremely. Tailor it for each job. If you’re applying to a telecom company, highlight telecom-related projects. Make it easy for recruiters to connect your experience to their needs.
Q16. What was your experience like as a data analyst?
I worked on BI tools, created dashboards, conducted requirement analysis, handled ETL pipelines, and sometimes even interacted directly with clients. It gave me a broad understanding of data handling.
Q17. Where can learners find projects for practice?
There are tons of open-source projects available online. Platforms like Kaggle, LinkedIn groups, and even AI tools like ChatGPT can help generate project ideas. Concentrate on sectors such as banking, telecommunications, and healthcare.
Q18. Is there any age restriction for entering data science or analytics?
Not at all. Age is not a barrier. However, entry-level data scientist roles can be harder to get, so many people—including myself—start as analysts and then transition.
Q19. Is the combination of Python, Power BI, and SQL sufficient for a data role?
Definitely. If you’re proficient in these three, you can land strong entry-level opportunities in data analytics.
Q20. What are the common topics required for both analysts and scientists?
The overlap includes Python and SQL. While some analyst roles may not require ML, a good grasp of data handling, visualization, and problem-solving is essential for both.
Q21. What advice would you give to aspiring data scientists?
Stick to the course material provided by your institute, practice Python programming, understand statistics, and master machine learning techniques like encoding and scaling. Real practice is key.
Refer these articles:
- From Physics Graduate to Data Scientist: Gujuri's Inspiring Journey
- Ayush Mehta’s Journey from Engineer to Data Scientist
- Mechanical Engineer to Data Scientist: The Journey of Vishwas
Valuable Lessons from Yash Sahindrakar's Data Science Journey
- Discover inspiring insights and practical tips from Yash Sahindrakar’s path to success in data science.
- Yush transitioned from mechanical engineering to IT/data science due to job scarcity.
- Datamites helped spark his interest and provided quality teaching.
- Started with no prior programming knowledge.
- Career progression: Software Engineer → Data Analyst → Data Scientist.
- Focus on Python, SQL, Excel fundamentals before machine learning.
- Interviews focused on Python, data structures, and project experience.
- Used HackerRank and LinkedIn groups for practice and updates.
- Daily tasks include Python coding, AWS Lambda, cybersecurity projects, and occasional UI work.
- Uses AWS services, Power BI, and company-specific BI tools.
- Strong Python coding skills essential for data scientists.
- Machine learning, AI, SQL are also critical skills.
- LinkedIn is key for networking and job searching.
- Tailored resumes with domain projects improve job chances.
- Age is not a barrier; entry via data analyst role suggested.
- Python, SQL, Power BI sufficient for beginners to get started.
- Data analysts and scientists both need Python/SQL; scientists need deeper ML knowledge.
- Freshers face practical coding tests; experienced candidates might not.
- Banking, telecom, healthcare are high-demand industries.
- Large companies have data engineers for data collection; startups expect analysts to do it.
- Cloud/infrastructure professionals should start as analyst/engineer before becoming a scientist.
- Strong fundamentals and live coding practice improve interview success.
- Preparation time ranges 3-6 months based on background.
Refer these articles:
Yash’s journey from a mechanical engineering graduate to a data scientist is proof that with the right mindset, strategic learning, and proper guidance, anyone can enter the tech world. His story also reinforces how platforms like DataMites can act as a launchpad for career transformations.
If you're a professional contemplating a similar shift or a student eyeing data science as a future, remember: foundational skills, consistency, and networking are your greatest tools.
If you’re considering a move into data science, now is the perfect time to upskill. The global data science platform market was valued at USD 15.2 billion in 2024 and is projected to reach USD 144.9 billion by 2033, growing at a compound annual growth rate (CAGR) of 27.08% between 2025 and 2033, according to the IMARC Group. This significant growth is driven by the rising volume of digital data generated across multiple industries. To stay competitive in this dynamic field, it’s crucial to choose a training institute that offers hands-on learning, real-time internships, and strong placement support. Enrolling in a reputed data science training in Bangalore, Hyderabad, Chennai, Mumbai, or Delhi can be your gateway to a successful tech career.
DataMites has established itself as a top provider of training in Data Science, AI, Machine Learning, Python Development, and Data Analytics. Accredited by IABAC and NASSCOM FutureSkills, DataMites delivers expert-led training, real-world project experience, and dedicated placement assistance, helping learners transition smoothly into data science careers.
With offline data science courses in Bangalore, Hyderabad, Chennai, Pune, Ahmedabad, Coimbatore, and Mumbai, alongside flexible online courses, DataMites ensures accessible learning for individuals from diverse backgrounds. Its practical, industry-aligned curriculum prepares freshers, working professionals, and career changers with the skills necessary to excel in data-driven roles.