What is Data Science in Simple Words?
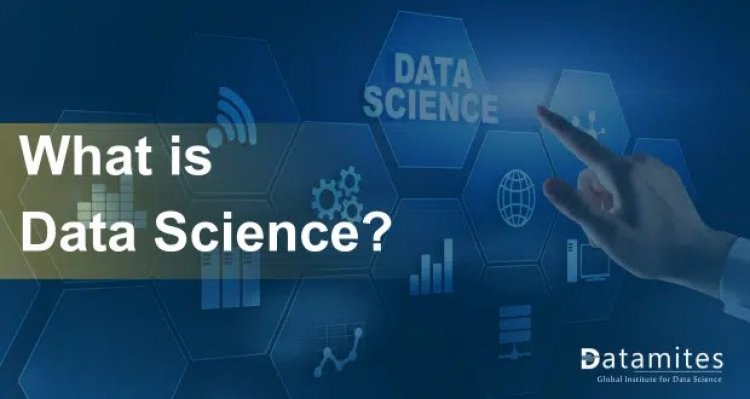
In today’s data-driven world, the term “Data Science” has become increasingly prevalent. It’s a buzzword that’s often thrown around in conversations about technology, business, and innovation. But what exactly is data science, and why is it so important? In this article, we’ll break down the concept of data science in simple terms, helping you understand its significance and how it impacts our lives.
Understanding Data Science: A Comprehensive Overview
At its core, data science is the field that deals with extracting valuable insights and knowledge from data. It combines various techniques from mathematics, statistics, computer science, and domain expertise to analyse and interpret complex data sets.
According to a Precedence Research report, the worldwide market size for data science platforms was valued at USD 112.12 billion in 2022. Projections indicate that by 2032, this market is anticipated to expand to approximately USD 501.03 billion. This growth trajectory suggests an expected compound annual growth rate (CAGR) of 16.2% from 2023 to 2032.
Data scientists use these insights to solve real-world problems, make informed decisions, and drive business growth.
Read the following articles:
- How to Become A Data Scientist
- Difference Between Data Scientist and Data Engineer
- Difference Between Data Science and Data Analytics
The Data Science LifeCycle
The Data Science LifeCycle typically involves several key stages:
- Problem Identification: Define the problem or question to be addressed.
- Data Collection: Gather the necessary data from various sources.
- Data Cleaning and Preprocessing: Clean and format the data to remove errors or inconsistencies.
- Exploratory Data Analysis (EDA): Perform initial analysis to understand the data’s characteristics, patterns, and anomalies.
- Feature Engineering and Selection: Create and select the most relevant features (variables) for the analysis.
- Model Building: Develop predictive or descriptive models using statistical or machine learning techniques.
Significance of Data Science in Today’s World
Data science has become increasingly important in today’s world due to its ability to turn large volumes of complex data into insights and knowledge that can drive decision-making. By applying techniques from areas such as statistics, machine learning, and predictive analytics, data scientists can uncover patterns and relationships within data that are not obvious.
According to a Fortune Business Insight report, the market for data science platforms is anticipated to experience robust growth, expanding from a valuation of $81.47 billion in 2022 to an estimated $484.17 billion by 2029. This growth trajectory represents a Compound Annual Growth Rate (CAGR) of approximately 29.0% during the forecast period.
Furthermore, data science plays a key role in advancing technological fields like artificial intelligence and the Internet of Things (IoT), enhancing the ability of machines to learn and make intelligent decisions. As the amount of data generated by humans and machines continues to grow exponentially, the importance of data science in extracting meaningful information and making informed decisions is more significant than ever.
The Brief History of Data Science:
The development of data science has been a long and complex process, marked by contributions from various fields including science, statistics, research, and computing. This timeline chronicles the progression of Data Science, charting its origins, application, and growing prominence throughout history.
1962 – 1992: The Foundation and Conceptualization Era
- Early 1960s: John Tukey began the conceptual shift in statistics towards data analysis, anticipating the integration of statistical methods and computer technology.
- 1974: Peter Naur uses the term “Data Science” in his work, setting a foundational definition for the field.
- 1977: Two significant developments:
- The International Association for Statistical Computing (IASC) was formed, emphasising the link between statistical methods, computer technology, and domain expertise.
- John Tukey’s second paper, “Exploratory Data Analysis,” highlights the importance of using data in hypothesis testing.
- Late 1980s – Early 1990s: The concept of Knowledge Discovery in Databases begins to take shape, leading to the first ACM SIGKDD Conference on Knowledge Discovery and Data Mining.
1992 – 2022: Expansion, Technological Advances, and Mainstream Integration
- 1994: Business Week’s cover story on “Database Marketing” signals the growing importance and challenges of managing large-scale personal data.
- 1999: Jacob Zahavi highlights the necessity for new tools to handle growing data sets, addressing challenges in data mining.
- 2001: Two major developments:
- The inception of Software-as-a-Service (SaaS), paved the way for cloud-based applications.
- William S. Cleveland’s action plan for data science education outlines six areas of study and emphasises the expansion of technical expertise.
- 2002: The launch of the Data Science Journal by the International Council for Science, focusing on data system descriptions, internet applications, and legal issues.
- 2006: Release of Hadoop 0.1.0, a significant advancement in processing and storing big data.
- 2008 – 2012: The term “data scientist” became popularized by DJ Patil and Jeff Hammerbacher. Harvard University later labelled it the sexiest job of the 21st century.
- 2009: NoSQL gains prominence, led by Johan Oskarsson’s discussions on open-source, non-relational databases.
- 2011: A 15,000% increase in job listings for data scientists; the concept of data lakes was introduced by James Dixon.
- 2013: IBM’s report shows a massive increase in data generation, with 90% of the world’s data created in just two years.
- 2015: Significant advancements in AI and deep learning, evidenced by Google’s improvements in speech recognition and the widespread adoption of AI in software projects.
This overview captures the evolution of data science from its early conceptual stages to its current status as a crucial component in technology and business
What is data science used for?
Data science uses scientific methods, processes, algorithms, and systems to extract knowledge and insights from structured and unstructured data. It is widely used for a variety of purposes across different industries and sectors. Some of the key applications include:
- Data science is used for a variety of purposes, including:
- Predictive Analytics: Forecasting future trends and behaviors using historical data.
- Decision Making: Assisting in making informed business decisions based on data analysis.
- Pattern Recognition: Identifying patterns and trends in large datasets.
- Machine Learning: Developing algorithms that improve automatically through experience and data.
- Customer Insights: Understanding customer behaviours and preferences to enhance user experience.
- Fraud Detection: Identifying unusual patterns that could indicate fraudulent activities.
- Risk Management: Evaluating and managing potential risks in business operations.
Read the article : Top IT Companies in India
How does Data Science differ from other similar fields?
Data science is a multidisciplinary field that combines elements from several other fields, including statistics, computer science, and domain-specific knowledge. While it shares commonalities with these fields, there are distinct differences:
Data Science vs Data Analysis:
Depth: Data science is generally more in-depth than data analysis. It involves more complex processes like building predictive models and machine learning algorithms.
Tools and Techniques: Data scientists are expected to have a stronger background in coding and are often involved in creating more complex, automated processes. Data analysts use similar tools (like SQL, Python, or R) for simpler tasks like querying databases and performing basic statistical analyses.
Data Science vs Statistics:
Focus: Data science often focuses on making predictions and decisions based on data, utilising techniques like machine learning. Statistics is more about understanding and interpreting data, emphasising hypothesis testing and inference.
Methods: Data scientists frequently use automated methods to process large datasets and may prioritise practical effectiveness, whereas statisticians might focus more on the theoretical underpinnings of such methods.
Tools: Data scientists often work with programming languages like Python and R, and tools for handling big data. Statisticians might use similar tools but also rely heavily on more traditional statistical software.
Data Science vs Machine Learning:
Definition: Machine learning is a subset of data science focused on developing algorithms to learn from and make predictions or decisions based on data. Data science encompasses this but also includes data cleaning, exploration, and visualisation.
Application: Machine learning is more about developing models and algorithms, while data science covers the entire spectrum of data processing, from gathering and cleaning data to deploying machine learning models.
Understanding Data Science from a Well-known Thought Professor
Well-known professors from top universities across the globe have made significant contributions to the field of data science, each with their unique areas of expertise and research focus. Here’s a glimpse into what some of these thought leaders are working on:
- Dr. Shlomo Argamon (Illinois Institute of Technology): Dr. Argamon founded the Master of Data Science program at Illinois Institute of Technology. He has worked on applications in forensic linguistics, biomedical informatics, and researched topics like natural language style and individual reasoning.
- Dr. Magdalena Balazinska (University of Washington): An authority in big data science, Dr. Balazinska teaches computer science and engineering, with a focus on big data management, sensor and scientific data management, and cloud computing.
- Dr. Gaurav Bansal (University of Wisconsin, Green Bay): From a quality assurance executive to a professor, Dr. Bansal’s interests lie in data mining, trust, and internet security. He has been nominated for the Best Paper Award at the International Conference on Information Systems.
- Dr. William Bosl (University of San Francisco): Dr. Bosl is known for his healthcare research, especially using machine learning for early detection of neurodevelopmental disorders. He directs the master of science in health informatics program at the University of San Francisco.
- Dr. Tom Davenport (Babson College): A renowned professor in information technology and management, Dr. Davenport’s research spans analytics, business process reengineering, and knowledge management. He has authored several influential books on data science.
- Dr. David Donoho (Stanford University): Specialising in statistics, Dr. Donoho’s research includes data visualisation, harmonic analysis, and signal processing. He is known for contributing to the understanding of the maximum entropy principle and sparse data description.
- Dr. Yoav Freund (University of California, San Diego): An expert in machine learning, Dr. Freund co-directs the data science and engineering program at UC San Diego. His research spans bioinformatics, network routing, and computer vision.
- Dr. Fei-Fei Li (Stanford University): A prominent figure in AI and deep learning, Dr. Li contributed to the creation of the ImageNet database, pivotal in training AI systems in computer vision.
- Dr Andrew Ng (Stanford University): Renowned for his work in AI and machine learning, Dr Ng has been instrumental in projects like Google Brain and online education platforms like Coursera.
- Dr. Peter Norvig (Google Inc): A leading expert in AI, Dr. Norvig is known for his co-authorship of “Artificial Intelligence: A Modern Approach” and his work at NASA and Google.
Data Science Tools
Data science is a complex field, yet it’s made more approachable thanks to a variety of tools that aid data scientists in their work. Having explored the essence of data science and its lifecycle, let’s delve deeper into the tools that facilitate success in this domain.
- Python: A programming language renowned for its simplicity and readability, Python is widely used in data science for data analysis, visualisation, and machine learning. Its vast array of libraries like NumPy, Pandas, Scikit-learn, and Matplotlib makes it a favourite among data scientists.
- R: Another popular programming language in data science, R specialises in statistical analysis and graphical models. It’s highly extensible and has a variety of packages for data manipulation, calculation, and graphical display.
- SQL: Structured Query Language (SQL) is essential for data manipulation and retrieval from relational databases. It’s widely used for data extraction, transformation, and loading (ETL) processes.
- Jupyter Notebooks: An open-source web application, Jupyter Notebook allows you to create and share documents containing live code, equations, visualisations, and narrative text. It’s great for exploratory data analysis and visualisation.
- TensorFlow and PyTorch: These are open-source machine learning libraries for research and production. TensorFlow, developed by Google, and PyTorch, developed by Facebook, are both used for deep learning applications.
Data Science Skills
Data science is an interdisciplinary field that amalgamates a diverse range of skills and areas of knowledge. Here’s a brief overview of the essential skills:
- Statistical Analysis: Understanding statistical methods and techniques is crucial for interpreting data and making inferences.
- Programming: Proficiency in programming languages such as Python or R is vital for data manipulation, analysis, and machine learning.
- Data Manipulation and Analysis: Skills in handling, cleaning, and analysing large datasets are fundamental. This often involves using Python libraries like Pandas.
- Machine Learning: Understanding various machine learning algorithms and their applications is a key part of data science.
- Data Visualization: The ability to present data visually using tools like Matplotlib, Seaborn, or Tableau helps in making data understandable to non-technical stakeholders.
- Big Data Technologies: Knowledge of big data platforms like Hadoop or Spark can be important, especially when dealing with very large datasets.
Who is a Data Scientist and What Do They Do?
A data scientist is a professional who specialises in analysing, interpreting, and extracting meaningful insights from large sets of data. They use a combination of statistical, mathematical, programming, problem-solving, and data visualisation skills to turn complex data into information that can be used to make decisions.
The role of a data scientist typically involves several key responsibilities:
- Data Collection and Cleaning: Data scientists often start by collecting raw data from various sources. This data may be structured (like in databases) or unstructured (like text or images). They then clean and preprocess the data to remove inaccuracies and prepare it for analysis.
- Data Analysis: They use statistical methods and algorithms to analyse data. This can involve exploratory data analysis to discover patterns, trends, and relationships in the data.
- Machine Learning and Modeling: Data scientists frequently build predictive models using machine learning algorithms. These models can be used to forecast future trends or outcomes based on the data.
- Data Visualization and Communication: They present their findings in a way that’s understandable and actionable for decision-makers. This involves creating visualisations like graphs and charts and presenting insights clearly and concisely.
- Problem-Solving: Data scientists often work on complex problems and must be adept at finding solutions and making data-driven decisions.
What are the Challenges Faced by Data Scientists?
Data scientists face several challenges in their work, including:
- Data Quality and Availability: Ensuring data is clean, reliable, and relevant can be a major hurdle. Data scientists often spend significant time cleaning and preprocessing data to make it usable.
- Big Data Processing: Managing and processing large datasets, especially in real-time, can be technically challenging and resource-intensive.
- Algorithm Selection and Model Complexity: Choosing the right algorithms and models for specific problems is complex. Overly complex models can lead to overfitting, while overly simple models may underperform.
- Integrating AI and Machine Learning: Implementing AI and machine learning techniques to add value to the business can be difficult, especially in terms of scalability and integration with existing systems.
- Keeping Up with Rapid Technological Changes: The field of data science is evolving rapidly, making it necessary for data scientists to continually learn and adapt to new tools, techniques, and best practices.
- Ethical and Legal Issues: Ensuring compliance with data privacy laws and ethical standards in data usage is a critical responsibility.
What is the earning potential of a Data Scientist across the globe?
The earning potential of a Data Scientist varies significantly across different countries due to factors like the local economy, demand for data science skills, cost of living, and industry norms. Here’s an overview of the earning potential in the major countries:
- The average data scientist’s salary in the United Kingdom is £65,504 annually.
- The average data scientist’s salary in Canada is $107,046 a year.
- The average data scientist’s salary in the United States is $156,266 per year.
- The average data scientist’s salary in the United Arab Emirates is AED 141,000 annually.
- The average data scientist’s salary in Germany is €70,479 per year.
- The average data scientist’s salary in India is INR ₹14,00,000 annually.
- The average data scientist’s salary in South Africa is ZAR 649,181 annually.
- The average data scientist’s salary in Australia is AUD 128,500 yearly.
Data Science: The Future Ahead
As technology continues to evolve, data science will remain at the forefront of innovation. According to the U.S. Bureau of Labor Statistics, the domain of data science research is projected to experience a growth rate of 22% from the year 2020 to 2030.
Here are some trends to watch for:
- AI and Automation: Data science is becoming more automated, with AI-powered tools simplifying the process.
- Ethical Data Science: There will be a growing emphasis on ethical considerations, fairness, and transparency in data science.
- Edge Computing: Data processing at the edge (closer to where data is generated) will become more prevalent, enabling real-time analysis.
- AI in Healthcare: Expect significant advancements in healthcare, including AI-driven diagnostics and personalised medicine.
Data Science Example
Here are examples illustrating the versatility of data science in various fields:
- Agricultural Optimization: In Kenya, a small-scale farming community faced challenges in crop yields due to unpredictable weather patterns and soil quality. By implementing data science, they were able to analyse satellite imagery and soil data to make informed decisions about crop rotation, irrigation schedules, and fertiliser application. This led to a significant increase in crop yield and sustainability, showcasing how data science can revolutionise traditional farming methods.
- Urban Planning: The city of Barcelona utilised data science to enhance its urban infrastructure. By analysing traffic patterns, public transportation usage, and pedestrian flow through city-wide sensor data, they were able to redesign public spaces and transportation routes. This improved traffic congestion, reduced pollution, and enhanced the overall quality of life for its residents, demonstrating the impact of data science on urban living.
- Retail Customer Experience: A mid-sized retail chain in Canada employed data science to enhance its customer experience and business operations. Through the analysis of sales data, customer feedback, and supply chain logistics, they were able to optimise inventory levels, tailor marketing campaigns to customer preferences, and improve the in-store experience. This led to increased sales, customer satisfaction, and operational efficiency, highlighting data science’s role in retail industry transformation.
These examples reflect the broad and transformative applications of data science across different sectors and challenges.
Summary
In simple terms, data science is all about turning raw data into valuable insights. It’s a multidisciplinary field that uses mathematics, statistics, and computer science to uncover patterns, make predictions, and drive decision-making across various industries. Whether you’re a business leader, a student, or someone curious about the world of data science, understanding its fundamentals is increasingly important in our data-driven society. Embracing data science means embracing the future.
Data science empowers us to harness the potential of data, solve complex problems, and drive innovation in virtually every aspect of our lives. It’s not just a buzzword; it’s a fundamental tool for shaping the world around us.
DataMites Data Science Institute, recognized by the International Association of Business Analytics Certification (IABAC), provides an extensive range of courses in data science, encompassing both basic and advanced subjects, including artificial intelligence. This institute serves as a prime entry point for learning and networking with an international community of data science experts. For individuals fascinated by technology and business, choosing a data science career is a prudent choice. DataMites offers a robust foundation for embarking on this exciting career path, backed by its accreditation from IABAC.