What Recruiters Look for in Data Science Resumes
Unlock the secrets to crafting a standout data science resume that grabs recruiter attention. From technical skills to project impact, learn what truly matters. Discover tips to highlight your strengths and avoid common pitfalls. Perfect your resume and take a step closer to your dream data role.
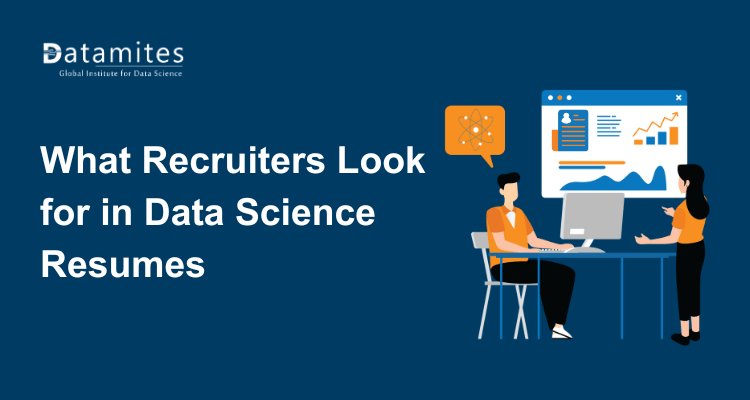
The demand for data scientists is rapidly increasing as organizations recognize the value of data-driven decision-making. This has created a competitive job market where standing out is crucial. Your data science resume needs to be more than a simple list of qualifications; it must be a compelling story that highlights your skills and potential to recruiters who are often overwhelmed with applications. Understanding what recruiters prioritize is essential for successfully navigating your career in data science, whether you are a recent graduate of a data science course or an experienced professional.
This blog will provide in-depth insights into the key elements recruiters look for in data science resumes. You will gain actionable strategies to craft a document that captures attention and effectively showcases your abilities. By understanding these crucial aspects, you can create a resume that opens doors to exciting opportunities in the expanding field of data science, playing a vital role in its future.
The Foundation: Structuring Your Data Science Resume
A well-structured resume is crucial for making a strong first impression in the competitive data science field, where hiring managers often face a deluge of applications. To stand out, your resume must be concise, clear, and optimized for both recruiters and Applicant Tracking Systems (ATS). Given the rapid expansion of the field, as highlighted by a Grand View Research report indicating that the global data science platform market was valued at USD 96.25 billion in 2023 and is projected to grow at a CAGR of 26.0% from 2024 to 2030, a well-organized resume is essential to showcase your qualifications effectively amidst this growth.
Key Principles of a Strong Data Science Resume
- Keep it concise – Ideally, a one-page resume works best for early to mid-career professionals, while senior professionals may extend to two pages.
- Present your most recent experiences first by using reverse chronological order.
- Ensure ATS compatibility – Many companies use ATS to filter applications before a human sees them. To pass this filter:
- Use commonly accepted fonts such as Arial, Calibri, or Times New Roman.
- Avoid fancy formatting, images, or graphics.
- Use bullet points instead of paragraphs.
- Include relevant keywords like "data science course," "data science training," "data science tools," etc.
- Focus on impact, not just responsibilities – Recruiters want to see how your contributions made a difference. Use quantifiable metrics to highlight your achievements.
- Customize your resume for each role— a one-size-fits-all approach won’t be effective. Emphasize the most relevant skills aligned with the job description.
Refer these articles:
- The Rise of Freelance Data Scientists: A Lucrative Career Option
- Top Data Science Trends to Watch in 2025
- Data Science and Automation: Will AI Replace Data Scientists?
The Must-Have Sections in a Data Science Resume
A comprehensive data science resume should include several key sections, each serving a specific purpose in highlighting your qualifications. Let's break down these essential components:
- Contact Information: Include your full name, professional email, phone number, and optionally, LinkedIn and/or a portfolio link. Omit unnecessary personal details.
- Professional Summary: Craft a concise "elevator pitch" highlighting your key data science skills, relevant experience, and career goals. Quantify achievements and tailor it to each specific job. Recent data science training graduates should emphasize academic accomplishments and projects.
- Skills Section: Categorize your hard skills (programming languages, tools, data visualization, ML, databases, cloud platforms) and soft skills (communication, problem-solving). List proficiency levels accurately.
- Work Experience: Detail previous roles with bullet points, emphasizing data science applications and quantifying your impact using metrics. Highlight relevant projects and transferable skills for career transitions.
- Education & Certifications: List degrees (reverse chronological), relevant coursework, and data science course or training details. Include certifications related to data science tools and methodologies. Completion of a data science course with placements is a significant highlight.
- Projects & Portfolio: Showcase practical data science skills through personal projects, competitions, or open-source contributions. Describe the problem, data, methods (including data science tools), and outcomes. Provide links to repositories like GitHub. This demonstrates hands-on expertise gained from a data science training institute or offline data science course.
Key Skills Recruiters Expect in Data Science Candidates
The landscape of skills in data science is constantly evolving, but certain core competencies remain highly sought after by recruiters.
- Programming Languages: Python, R, SQL: Proficiency in at least one of these languages is fundamental. Python is particularly popular due to its extensive libraries and versatility. R is widely used for statistical computing and analysis. SQL plays a crucial role in retrieving and managing database information. Many data science course curriculums heavily emphasize these languages.
- Tools & Libraries: Pandas, NumPy, Scikit-learn, TensorFlow, etc. Familiarity with relevant libraries and frameworks is crucial for data manipulation, numerical computation, machine learning, and deep learning. Recruiters look for candidates who can effectively utilize these tools to solve data-related problems.
- Data Visualization: Matplotlib, Seaborn, Tableau, Power BI: The ability to effectively communicate insights through visualizations is a critical skill in data science. Experience with tools like Matplotlib and Seaborn (in Python), as well as business intelligence platforms like Tableau and Power BI, is highly valued.
- Machine Learning & Statistical Modeling: A strong understanding of machine learning algorithms (regression, classification, clustering, etc.) and statistical modeling concepts is essential for many data science roles. Recruiters look for candidates who can not only apply these techniques but also understand their underlying principles and limitations.
- Data Wrangling & Cleaning: Real-world data is rarely clean and well-structured. The ability to effectively clean, transform, and prepare data for analysis and modeling is a fundamental skill. This often involves using tools and techniques learned in a comprehensive data science training.
- Communication and Storytelling with Data: Being able to translate complex technical findings into clear, concise, and actionable insights for both technical and non-technical audiences is a crucial skill. Recruiters look for candidates who can effectively communicate the "story" behind the data.
Refer these articles:
Crafting a Data-Driven Resume: The Power of Metrics
Treat your resume like a data-driven report; use metrics to showcase your impact. Instead of vague statements, quantify your achievements with numbers and percentages. For example, instead of "Improved model performance," specify "Increased model accuracy by 12% by implementing a new feature selection technique." This provides concrete evidence of your abilities and makes your accomplishments far more persuasive to recruiters.
Consider the scope of your projects, the efficiency improvements you implemented, or the positive business outcomes resulting from your analysis. Even when detailing projects from a data science course, strive to quantify your results. Demonstrating tangible achievements through metrics makes your resume significantly more compelling and highlights your ability to deliver measurable value.
Optimizing Your Resume for Targeted Data Science Roles
Generic resumes rarely succeed. Customizing your data science resume for each specific job is vital. Scrutinize the job description, identify key requirements, and prominently showcase your most relevant skills and experiences. For example, highlight NLP projects for NLP-focused roles. Strategically integrate keywords from the job posting to clear Applicant Tracking Systems (ATS) and grab recruiter attention.
Tailoring is significantly more effective; reports indicate a 65% higher interview rate for customized resumes. Understanding the unique demands of different data science career paths (e.g., data analyst, ML engineer) is therefore crucial for creating targeted and impactful applications
Common Mistakes That Can Ruin Your Data Science Resume
Avoid these common pitfalls to ensure your data science resume makes a positive impression:
- Typos and grammatical errors: Proofread meticulously! Mistakes indicate a lack of precision and carefulness.
- Generic language: Use specific examples and quantify your achievements.
- Too much jargon: While technical terms are necessary, ensure they are relevant to the role and avoid overly obscure acronyms without explanation.
- Lack of quantifiable results: As mentioned earlier, numbers speak volumes.
- Poor formatting and readability: Ensure your resume is well-organized and easy to scan.
- Irrelevant information: Focus on skills and experiences directly related to data science.
- Not tailoring to the job description: A generic resume shows a lack of specific interest in the role.
- Inconsistent formatting: Maintain a consistent style throughout the document.
Refer these articles:
How to Beat the Applicant Tracking System (ATS)
Many companies use Applicant Tracking Systems (ATS) to filter and rank resumes based on keywords and formatting. To ensure your resume is ATS-friendly:
- Use standard fonts: Stick to common fonts like Arial, Calibri, or Times New Roman.
- Avoid graphics, tables, and text boxes: These can sometimes be misinterpreted by ATS.
- Use clear and concise language: Avoid overly creative or complex phrasing.
- Incorporate relevant keywords: Carefully review the job description and include relevant skills and keywords throughout your resume.
- Use standard section headings: Label sections clearly (e.g., "Work Experience," "Skills," "Education").
- Submit your resume in the preferred format: Usually, this is a .doc or .pdf file.
Understanding how ATS works is crucial for maximizing your chances of your resume being seen by a human recruiter. Many best data science institute programs provide guidance on crafting ATS-friendly resumes.
Crafting a strong data science resume requires a strategic approach—understanding recruiter expectations, showcasing technical and soft skills, and maintaining a clear structure. To boost your chances, quantify your impact, tailor your resume, and avoid common mistakes. As AI transforms industries, recruiters seek experts in machine learning, analytics, and AI applications. Stay competitive by updating your resume and upskilling through a data science course in Hyderabad or offline training in Bangalore.
To stand out in this competitive field, aspiring data scientists should opt for hands-on training programs from reputable data science institutes in Bangalore, Hyderabad, Pune, Chennai, Ahmedabad, Coimbatore, Mumbai, Kolkata, and Delhi. Enrolling in a structured data science training institute with placement assistance and internship opportunities can give you a significant advantage when applying for jobs.
Among the top choices, DataMites Institute, ranked among India’s Top 20 AI Institutes by Analytics India Magazine (AIM) alongside IIT and IIM, offers industry-accredited Certified Data Scientist courses, certified by IABAC and NASSCOM FutureSkills. Their comprehensive curriculum ensures that candidates gain practical expertise in data science tools, machine learning models, and analytics techniques, making them highly desirable to recruiters.
For those preferring in-person learning, DataMites provides offline data science training in Hyderabad, Bangalore, Chennai, Pune, Ahmedabad, Coimbatore, and Mumbai, while also offering online courses for learners worldwide. Whether you're a fresher or an experienced professional, these programs help bridge the skill gap and prepare you for a successful data science career.
A well-structured data science resume highlights technical expertise, hands-on projects, and quantifiable impact while being optimized for ATS and recruiter readability. To improve your chances, consider enrolling in a data science training institute to gain relevant experience and certifications.
Ready to apply? Tailor your resume, highlight the right skills, and showcase your projects, and you'll be on your way to securing a top data science roles.