What Does a Data Scientist Do?
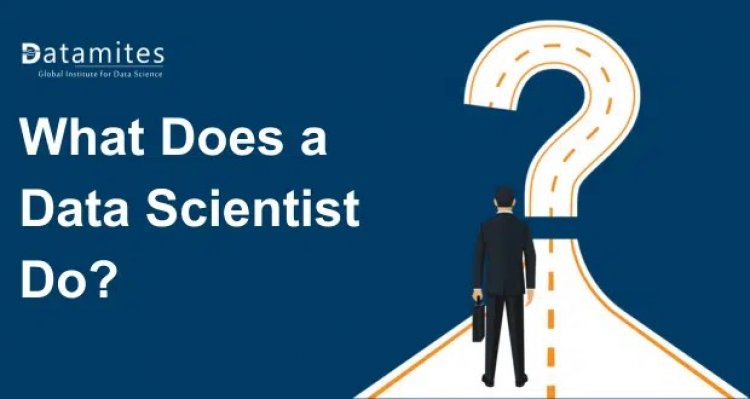
Data scientists are at the forefront of the modern technological era, wielding the power to turn vast and complex data sets into valuable insights and strategic guidance. In essence, they act as the alchemists of the digital age, transforming raw data into actionable intelligence. Their role encompasses a range of activities including data collection, cleaning, and analysis, as well as the application of advanced statistical methods, machine learning algorithms, and predictive modeling.
According to a report by Fortune Business Insights, the global market for data science platforms is projected to grow significantly, increasing from $81.47 billion in 2022 to $484.17 billion by 2029. This growth will be driven by a compound annual growth rate (CAGR) of 29.0% throughout the forecast period.
At the core of a data scientist’s work is the ability to ask the right questions, apply appropriate analytical techniques, and communicate findings effectively to stakeholders. They not only interpret data but also identify trends, patterns, and anomalies, using these insights to solve real-world problems, drive business decisions, and inform policy.
In this article, we will delve into the multifaceted role of data scientists, exploring their tools, techniques, and the impact they have across various industries.
Data Science Life-Cycle
The Data Science Life Cycle is a structured approach to managing and executing data science projects. It consists of several stages, each crucial for ensuring the effectiveness and success of a data science initiative. Here’s an overview of these stages:
Problem Definition: This initial phase involves understanding and defining the business problem. Data scientists collaborate with stakeholders to clarify objectives, determine the scope, and identify the key questions that need answering.
Data Acquisition and Preparation: Once the problem is defined, the next step is to gather relevant data. This includes sourcing data from internal systems, external databases, or public datasets. Data preparation involves cleaning and preprocessing the data to make it suitable for analysis.
Exploratory Data Analysis (EDA): In this stage, data scientists perform initial investigations on the data to discover patterns, spot anomalies, test hypotheses, and check assumptions using statistical summaries and visualizations.
Model Development: Here, data scientists select and apply various statistical models and machine learning algorithms. The choice of model depends on the problem type (e.g., classification, regression) and the nature of the data.
Model Training and Testing: The chosen models are trained on a subset of the data, and their performance is evaluated using various metrics. This stage often involves a process of tuning and refinement to improve the model’s accuracy and effectiveness.
Unveiling the World of a Data Scientist
A data scientist is a professional who uses their expertise in data analysis, statistics, and programming to extract valuable insights and knowledge from large datasets. They employ various tools and techniques to clean, process, and analyze data, ultimately helping organizations make data-driven decisions, solve complex problems, and identify trends and patterns that can drive business growth and innovation.
Data scientists play a crucial role in fields such as business, healthcare, finance, and many others by harnessing the power of data to inform and optimize decision-making processes.
Refer these articles:
- What Separates Data Analytics from Data Science
- Data Scientist and Data Engineer Distinctions
- 10 Myths about Data Science
Exploring the Work of a Data Scientist
The Data Scientists primary goal is to turn raw data into actionable insights, which can inform decision-making, solve problems, and drive business or research objectives. Here are the key tasks and responsibilities of a data scientist:
Data Collection: Data scientists gather and collect data from various sources, which may include databases, APIs, websites, sensors, or other data repositories. They ensure data quality and completeness.
Data Cleaning and Preprocessing: Raw data is often messy and may contain errors or missing values. Data scientists clean and preprocess the data to make it suitable for analysis. This involves tasks like imputing missing values, removing outliers, and standardizing data formats.
Exploratory Data Analysis (EDA): EDA involves visualizing and summarizing data to understand its characteristics, identify patterns, correlations, and anomalies. Data scientists use various statistical and data visualization techniques for this purpose.
Data Modeling: Data scientists build predictive and descriptive models using machine learning, statistical analysis, or other data analysis techniques. These models can help solve specific problems, make forecasts, or classify data points.
Feature Engineering: Feature engineering is the process of creating new features or transforming existing ones to improve the performance of machine learning models. Data scientists select and engineer features to make models more effective.
Model Training and Evaluation: Data scientists train machine learning models using historical data and evaluate their performance using metrics like accuracy, precision, recall, and F1-score. They fine-tune models to improve their predictive capabilities.
Data Visualization: Effective data visualization is crucial for communicating insights to stakeholders. Data scientists create charts, graphs, and dashboards to present findings in an understandable and actionable way.
Interpretation and Insight Generation: Data scientists analyze model results and draw meaningful conclusions from the data. They identify trends, patterns, and insights that can be used to inform decision-making.
Deployment: In some cases, data scientists are responsible for deploying machine learning models into production systems to automate decision-making or provide real-time predictions.
Continuous Learning and Improvement: The field of data science is continually evolving, and data scientists must stay updated with the latest techniques and tools. They may also revisit and update models as new data becomes available.
Why Become a Data Scientist?
Becoming a data scientist can be a highly rewarding career choice for several reasons:
High Demand: The field of data science is growing rapidly, with businesses and organizations increasingly relying on data-driven decision-making. This growth translates into a high demand for skilled data scientists across various industries.
Lucrative Salaries: Due to the high demand and the specialized skill set required, data scientists often command high salaries. The exact amount can vary based on location, experience, and the specific industry, but generally, data science is known to be a well-paying career.
Impactful Work: As a data scientist, you have the opportunity to make a significant impact on your organization. Your insights can lead to better business decisions, innovative products, and improved customer experiences.
Intellectual Challenge: The field is intellectually stimulating. Data scientists regularly solve complex problems and are at the forefront of developing new methods and technologies in data analysis, machine learning, and artificial intelligence.
Versatility: Data science skills are versatile and can be applied in many different sectors such as healthcare, finance, technology, government, and more. This versatility allows for a wide range of career opportunities and paths.
The Demand for Data Scientists or Data-Driven Industries: Adapting to Data Science
Data Scientist Demand remains strong in various industries as organizations continue to recognize the value of data-driven decision-making. Here are some key points about the demand for data scientists by industry:
Technology: Tech companies, including giants like Google, Facebook, and Amazon, are constantly in need of data scientists to improve algorithms, enhance user experiences, and optimize products.
Finance: The financial sector relies on data scientists for risk assessment, fraud detection, algorithmic trading, and customer analytics.
Healthcare: Healthcare organizations use data scientists to analyze patient data, improve treatment outcomes, and develop predictive models for disease diagnosis and prevention.
Retail: Retailers leverage data scientists to optimize pricing strategies, personalize marketing campaigns, and enhance supply chain management.
Manufacturing: Data scientists in manufacturing help streamline operations, reduce production costs, and predict maintenance needs through predictive analytics.
Telecommunications: Telecom companies use data scientists for network optimization, customer churn prediction, and improving service quality.
Energy: The energy sector employs data scientists to optimize resource allocation, monitor equipment performance, and develop renewable energy solutions.
E-commerce: E-commerce companies rely on data scientists for recommendation systems, customer segmentation, and fraud detection.
Government: Government agencies use data scientists for policy analysis, urban planning, and to improve public services.
Consulting: Consulting firms across industries hire data scientists to offer data-driven insights and solutions to their clients.
The Other Data Science Career Paths: An Overview
A career in data science offers a variety of paths, each catering to different interests and skill sets within the field. Here’s an overview of some key data science career paths:
Data Analyst: This role involves analyzing data to help businesses make more informed decisions. Data analysts typically work with large data sets, use statistical techniques, and present findings clearly and understandably. Skills often include SQL, Excel, basic statistical knowledge, and data visualization tools like Tableau or PowerBI.
Machine Learning Engineer: These professionals specialize in building and deploying machine learning models. They are more focused on the software engineering side of data science, often requiring strong skills in programming, algorithm development, and system design.
Data Engineer: Data engineers build and maintain the systems that allow data to be accessed and used effectively. They work on the architecture for data generation, storage, and management. Key skills include database management, ETL (extract, transform, load) processes and big data technologies like Hadoop and Spark.
Business Intelligence Analyst: BI Analysts focus on analyzing data to find insights related to business performance. They often create dashboards and reports for business leaders. Skills in SQL, data visualization, and an understanding of business processes are crucial.
Research Scientist: These are often PhD-level professionals working in more theoretical aspects of machine learning and artificial intelligence. They focus on developing new methods and algorithms, often in academic or specialized industrial research settings.
Data Architect: Data architects design and manage an organization’s data infrastructure. They ensure that the data ecosystem is scalable, secure, and serves the needs of various stakeholders.
What are the qualifications and skills required to become a Data Scientist?
Becoming a data scientist typically involves a combination of formal education, technical skills, and soft skills. Here’s a breakdown:
Qualifications for a Data Scientist:
Educational Background:
- Bachelor’s degree in computer science, statistics, mathematics, or related fields.
- Advanced degrees (Master’s or PhD) are often preferred, especially in specialized or technical areas.
Technical Proficiency:
- Proficiency in programming languages like Python, R, or Java.
- Experience with database management and SQL.
Statistical Knowledge:
- Strong foundation in statistics and probability.
Machine Learning:
- Understanding of machine learning algorithms and principles.
Data Management:
- Skills in handling and processing large datasets.
Certifications:
- Data Science Certification, big data, or specific tools and technologies can be advantageous.
Skills Required to Become a Data Scientist:
Analytical Skills:
- Ability to analyze complex data and draw meaningful insights.
Problem-Solving Skills:
- Strong problem-solving abilities to devise data-driven solutions.
Communication Skills:
- Ability to communicate complex data insights clearly to non-technical stakeholders.
Critical Thinking:
- Critical thinking and scepticism when working with data and making inferences.
Data Visualization:
- Skills in data visualization tools like Tableau, PowerBI, or Matplotlib in Python.
Mastering Data Science: Popular Certification Providers in the Digital Era
Several reputable organizations and platforms provide data science certification. Keep in mind that the effectiveness and recognition of certification often depend on your specific career goals and the industry you’re targeting. Here are some well-known data science certification providers:
- IBM Data Science Professional Certificate
The IBM Data Science Professional Certificate provides a robust curriculum to establish a solid foundation in data science. Through this program, you will acquire essential skills and knowledge to excel in the dynamic field of data science.
- Microsoft Certified: Azure Data Scientist Associate
Azure Data Scientist Associate certification, presented by Microsoft, is dedicated to honing expertise in data science with a specific emphasis on leveraging the Azure platform. Obtain this certification to showcase your proficiency in applying advanced data science techniques within the Azure ecosystem.
- Google Cloud Certified: Professional Data Engineer
Provided by Google Cloud, this certification is for individuals who design and build data processing systems and create machine learning models on Google Cloud Platform.
- IABAC: Certified Data Scientist
IABAC provides the Certified Data Scientist Certification, which attests to your advanced skills in data analysis, machine learning, and predictive modeling.
Renowned as a leading certification in the realm of data science, the Certified Data Scientist (CDS) is widely acknowledged and respected. With an impressive roster of over 30,000 active professionals who have attained this esteemed certification, it stands as a testament to its prominence and credibility in the field.
- PeopleCert: Data Science Certification
Explore the PeopleCert Data Science Certification Suite, meticulously crafted and evaluated by global industry experts. This comprehensive suite equips you with the essential skills and knowledge to comprehend and apply data science concepts, terms, and tools effectively in real-world business scenarios.
Popular University that offers a Data Science Program
There are several popular universities around the world known for offering excellent Data Science programs. Here are a few notable ones:
- Stanford University: Known for its cutting-edge research and strong emphasis on practical applications of data science, it serves as a global hub for fostering innovation and collaboration in the rapidly evolving field of technology and information sciences.
- Massachusetts Institute of Technology (MIT): Offers robust data science programs with a strong foundation in mathematics and computer science, empowering students to tackle complex real-world challenges through interdisciplinary approaches and hands-on research experiences.
- University of California, Berkeley: Known for its interdisciplinary approach, combining statistics, computer science, and data analysis, it cultivates a dynamic learning environment where students engage with cutting-edge technologies and contribute to groundbreaking advancements in the field of data science.
- Harvard University: Offers data science programs through its Institute for Applied Computational Science, emphasizing real-world applications, and fosters a collaborative learning community where students not only acquire technical expertise but also develop critical thinking skills to address societal challenges through data-driven solutions.
- Carnegie Mellon University: Renowned for its strong focus on machine learning and artificial intelligence within data science, stands at the forefront of pioneering research and innovation in shaping the future of intelligent technologies.
- University of Oxford: Combines rigorous statistical methodology with practical data analysis, fostering a dynamic learning environment where students engage in cutting-edge research to address real-world challenges.
- ETH Zurich: Known for its strong mathematical foundation and research opportunities in data science, cultivates a collaborative and interdisciplinary atmosphere, empowering students to bridge theory and application in the rapidly evolving field of data-driven innovation.
- Imperial College London: Offers a blend of theoretical and practical aspects of data science, emphasizing big data and analytics, and provides students with access to state-of-the-art technology and industry partnerships, ensuring a comprehensive and industry-relevant education in the field.
- National University of Singapore (NUS): Recognized for its focus on the integration of technology and data science, NUS fosters a global perspective, preparing students to address complex challenges by combining cutting-edge advancements in both fields for innovative solutions.
- Tsinghua University: Leading in data science education in China, with a strong emphasis on research and innovation, Tsinghua’s commitment to cultivating entrepreneurial skills equips graduates to drive advancements and contribute to the evolving landscape of data-driven industries globally.
Global Earning Potential of a Data Scientist
The global salary for data scientists has surged in response to the increasing demand for their expertise.
- The average data scientists salary in the United Kingdom is £65,504 annually.
- The average data scientists salary in Canada is $107,046 a year.
- The average data scientists salary in the United States is $156,266 per year.
- The average data scientists salary in the United Arab Emirates is AED 141,000 annually.
- The average data scientists salary in Germany is €70,479 per year.
- The average data scientists salary in India is INR ₹14,00,000 annually.
- The average data scientists salary in South Africa is ZAR 649,181 annually.
- The average data scientists salary in Australia is AUD 128,500 yearly.
Key Influences on Data Scientist Compensation
Several factors can influence a data scientist’s salary:
- Experience: More years of experience typically result in higher salaries.
- Education: Advanced degrees like a master’s or PhD can command higher pay.
- Location: Salaries vary significantly based on the cost of living in different regions.
- Industry: Certain industries, like tech or finance, tend to offer higher salaries.
- Company size: Larger companies often pay more than smaller ones.
- Skills and specialization: Specialized skills, like machine learning or deep learning, can lead to higher pay.
What is the future scope of a Data Scientist?
The future scope of a Data Scientist remains promising as businesses increasingly rely on data-driven decision-making. With the growing volumes of data generated, there will be a continued demand for professionals who can extract valuable insights, develop predictive models, and drive innovation.
As per the U.S. Bureau of Labor Statistics, the field of data science research is anticipated to witness a 22% increase in employment opportunities from 2020 to 2030.
As AI and machine learning technologies advance, data scientists will also play a crucial role in harnessing their potential. Additionally, industries such as healthcare, finance, and cybersecurity will offer exciting opportunities for data scientists to make a significant impact.
Read the article: The Best IT Firms in India
Wrap Up:
A data scientists role revolves around harnessing the power of data to solve real-world problems and inform strategic decisions. They employ a combination of technical skills and analytical thinking to uncover hidden patterns and trends within data, making them invaluable in today’s data-driven world. Students should consider this career path as it offers not only a promising job market with competitive salaries but also the opportunity to work on cutting-edge projects, contribute to advancements in technology, and have a significant impact on various industries, from healthcare to finance and beyond.
DataMites Data Science Institute, accredited by the International Association of Business Analytics Certification (IABAC), provides a comprehensive range of data science courses that encompass both fundamental and advanced subjects, including artificial intelligence. This institution serves as an excellent entry point for individuals seeking to acquire knowledge and establish connections within the global data science community. For those who have a strong interest in the convergence of technology and business, embarking on a data science career is a strategic choice. With its IABAC endorsement, DataMites offers a robust educational base to initiate a rewarding career in this dynamic field.