What is Predictive Analytics?
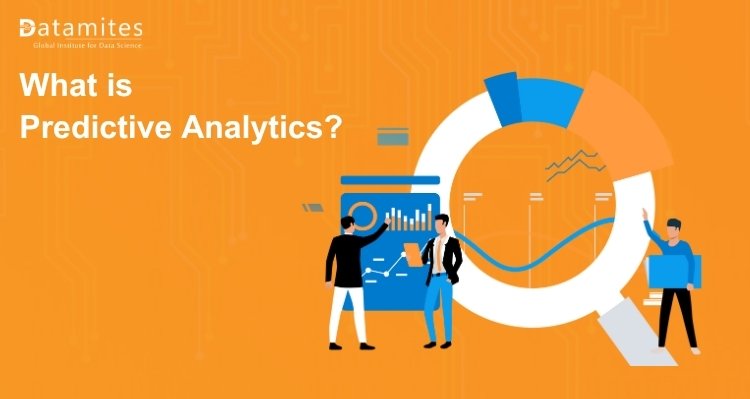
In the current environment, the adage “survival of the fittest” is by no means inaccurate. Each company has rivals, and for survival and expansion, each one must continue operating despite fierce opposition.
This scenario needs to be analyzed, and for so enough and pertinent data are needed. Big data is fortunately readily available, saving the day. Planning appropriate measures for goal achievement at the appropriate moment can be aided by analysis of recent and previous pertinent data. Predictive analytics is a crucial subset of data analytics since it relies on a variety of complex factors and can support other phases of analytics. Predictive analytics provide knowledge that can help other analytics sections provide the right inputs for the success of a firm as a whole. Businesses can utilize predictive analytics to find out information about customer attrition, how to improve marketing plans, what customers expect, and other issues.
In this article, we will go into great detail regarding predictive analytics including its types, techniques, and benefits of predictive analytics.
What Is Predictive Analytics?
In order to evaluate risk, project future business trends, and foresee when maintenance is necessary, many companies utilize predictive analytics, a crucial analytical method. While looking for patterns and trends in historical data, data scientists use a variety of regression models and machine learning approaches.
Predicting future events with a high degree of accuracy is the fundamental aim of predictive analytics. This distinguishes predictive analytics from prescriptive analytics, which use optimization techniques to find the best solutions to address the trends revealed by predictive analytics, and descriptive analytics, which aids analysts in assessing what has already happened.
Refer these articles:
- 5 Reasons, Why One Should Learn Data Analytics
- Data Science vs. Big Data vs. Data Analytics
- Data Science Vs Data Analytics
Types Of Predictive Analytics Models
Predictive analytics models are developed to analyze historical data, identify patterns, track trends, and use that knowledge to forecast future trends. Predictive analytics models that are commonly used are classification, clustering, and time series models.
- Classification model- Supervised machine learning models include classification models as a subset. Based on historical data, these models classify data and describe relationships within a specific dataset. This strategy, for instance, might be applied to the segmentation of clients or prospects by categorizing them into categories. Other uses for this include fraud detection and credit risk assessment; it can also be used to respond to inquiries with binary outputs, such as answering true or false or yes or no. There are several different categories of categorization models, including logistic regression, decision trees, random forests, neural networks, and Naive Bayes.
- Regression model- When attempting to estimate a numerical value, such as the amount of time a target audience will spend considering an airline reservation before making a purchase decision or the amount of money a person would spend on car payments over a specific period of time, the organization must use a regression method.
- Clustering model- Clustering models are an example of unsupervised learning. They classify data according to comparable qualities. An online store, for example, could use the approach to categorize customers into groups with similar characteristics and then create marketing plans for each group. Examples of common clustering techniques include K-means, mean-shift, density-based spatial clustering of applications with noise (DBSCAN), expectation-maximization (EM) clustering with Gaussian Mixture Models (GMM), and hierarchical clustering.
- Outliers model- The outliers model focuses on typical values that are aberrant in a dataset, that is, they are completely out of alignment with or remote from the majority of the other values. For instance, a comic book is an outlier that is simple to spot in a data collection of fiction books. The financial industry relies heavily on this model to identify counterfeit or fraudulent transactions involving transfers of funds, loans, and insurance claims.
- Forecast model- One of the most popular models for predictive analytics is forecast model. Based on previous data, this model aims to calculate the numerical value of fresh data. Anything that has historical numerical data can use this model.
- Time series model- Different data inputs are used in time series models at predetermined time intervals such as daily, weekly, monthly, and so on. It is common practice to plot the dependent variable over time when analyzing data for seasonality, trends, and cyclical behavior. This can aid in identifying the need for specific model types and transformations. The autoregressive (AR), moving average (MA), ARMA, and ARIMA time series models are examples. A call center, for example, might use a time series model to forecast how many calls it will receive per hour at different times of day.
Use Of Predictive Analysis In Business
There are many diverse uses for predictive analysis. Models can be used by businesses to further their objectives and enhance operations. Businesses commonly utilize predictive models to enhance their outreach and customer service.
This form of statistical analysis can be used by executives and business owners to understand consumer behavior. For instance, a business owner can utilize predictive approaches to pinpoint and identify loyal clients who may leave and patronize a rival.
Advertising and marketing heavily rely on predictive analytics. Models can be used by businesses to identify which clients are most likely to respond favorably to marketing and sales initiatives. Instead of conducting blanket advertising, business managers can save money by focusing on clients who will respond favorably.
Refer these articles:
- How to Become a Data Analyst in India
- How much is the Data Analytics course fee in India?
- Data Analytics Course Fees in Bangalore
- Data Analytics Course Fees in Chennai
Benefits Of Predictive Analytics
Predictive analytics improves the accuracy and dependability of peering into the future.. Models can be used by investors, financial experts, and company executives to assist lower risk. For instance, by taking certain criteria into mind, such as age, capital, and aspirations, an investor and their advisor can utilize certain models to help create an investment portfolio with minimal risk to the investor.
When models are utilized, cost savings is significantly impacted. Businesses can predict whether a product will succeed or fail before it is released. Alternatively, they can use predictive approaches to set aside money before the manufacturing process even starts for production improvements.
Certified Data Analyst Course
End Note
In all kinds of enterprises, predictive analytics has emerged as a crucial activity for survival and expansion. It is therefore essential to comprehend its advantages before accepting it and putting it into practice.
If you’re interested in learning more about the fascinating subject of data analysis, consider enrolling in a certified data analyst course. Gain valuable skills in statistical analysis, data visualization, and decision-making. Boost your career prospects with in-depth training and certification in this dynamic and rapidly growing field.
DataMites is a leading player in the field of data analytics, offering comprehensive training programs through their esteemed institute. With a focus on hands-on learning and real-world applications, DataMites empowers individuals with the skills needed for a successful career in data analytics. Join DataMites training institute for expert guidance and practical insights.
Data Science vs Data Analytics