What role does Data Science play in Retail?
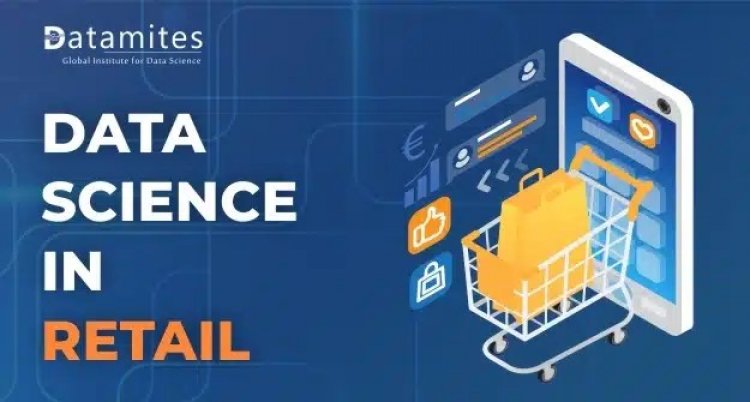
In today’s world, data is the engine that powers every company. The potential benefits of the data are being pursued by many significant organizations from various industries. Thanks to the solutions that data scientists have offered, several economic sectors are undergoing a fundamental revolution.
As tech behemoths like IKEA, Amazon, and Netflix already make use of all potential advantages, the application of data science in the retail industry has increased as well. In India, the retail industry is expected to reach a whooping height of US$ 2 trillion by the year 2032, according to a survey held by the Boston Consulting Group.
Data Science in Retail – Revalorizing the Shopping Experience
There is too much potential for income and growth for retailers and consumer goods companies in particular, in this data-driven world than can be ignored. Customers now demand more individualized experiences when they enter a store and expect to be able to shop 24 hours a day, across numerous online and offline channels.
Retail enterprises, and all businesses, in general, continue to face an upheaval that intensifies the need for retailers to become more customer focused and data-driven than ever before. Retailers undoubtedly think that a metaverse strategy is something they need. It has swiftly become a cornerstone of brand positioning as consumers connect with brands more frequently online. By 2026, the market for data science platforms is projected to reach $322.9 billion, expanding at a compound annual growth rate (CAGR) of 27.7%. Big data‘s rising popularity and the widespread use of cloud-based services are driving this expansion.
In a commercial setting, data serves as the foundational material from which knowledge is formed. As a result, business people looking to make wise decisions now have access to a greater variety of information. Furthermore, thorough analysis of enormous volumes of data can be used to influence or even manipulate consumer decisions. Data science in retail tailors helps make marketing strategies and enhances supply networks for retailers thereby bringing about better customer service and higher revenues.
Retailers have a fantastic chance to use data science to transform the customer data they already have into useful insights that will ultimately increase sales. Let’s get to know how data science helps retailers one by one;
- Recommending systems
Recommendation systems are generally those that anticipate user preferences while they are browsing the Internet and filter the information. It has been shown to be a fantastic tool for retailers to anticipate consumer behaviour. Customers are able to spot patterns and boost their sales, which in turn increases revenue, thanks to recommendations.
While there are many different aspects to personalization, businesses will have the chance to choose the content, goods, and promotions that are offered to customers at various stages of their customer experience. Retailers may now choose the best products to present by taking into account both larger trends and previous client feedback.
Refer to the article: Using Data Science at every step of the Business way
- Price optimization
Having the proper price for both the client and the retailer is a big benefit provided by optimization techniques. With the aid of a real-time optimization model, shops can draw in clients, hold their interest, and implement personalised pricing strategies. The data collected from various sources can be examined to identify the pricing flexibility, the customer’s location, their purchasing behaviour, the time of year they make their purchase, and the pricing of the competition.
While retailers have historically utilized price optimization to change the costs of a few key value items, retail data science now enables much more sophisticated and dynamic price modifications depending on demand, competition, economics, and other factors. This strategy not only offers consumers fair prices on the goods they are most interested in, but it can also enable merchants to more strategically set prices based on their priorities.
- Identifying fraud
Financial losses due to fraud is a common problem in every country. Both consumers and retailers are impacted by credit risks, financial losses, and delivery fraud. The traditional method of detecting fraud is rule-based, and it essentially boils down to a race between the criminals’ methods and the seller’s fraud detection system. Whereas, the current methods use the enormous quantity of data gathered from internet transactions to predict fraudulent transactions, something the conventional method cannot do.
Truth be told, with digitization and online transactions, fraud dangers have risen in recent years. As a result of such behavior, customers lose faith in businesses. Data science may be useful in this situation for the retail industry. Data science helps retailers sustain their reputation in the marketplace by safeguarding their key information. Companies are now turning to machine learning and data science as they continue to face financial challenges. They can now continuously monitor all actions and stop any fraudulent transactions thanks to data science.
- Cross-selling and upselling techniques that are wise
To increase their revenue in the retail sector, all businesses engage in cross-selling and upselling. The practice of cross-selling involves advising clients to purchase complementary goods. Upselling, on the other hand, refers to the technique of offering clients the chance to purchase a high-end product that is superior to the one they are contemplating.
Read this article: Role of Data Science in Making Education A Lot Better
- Merchandising
The practice of merchandising has grown crucial to the retail industry. This idea encompasses a sizable portion of the actions and tactics used to boost sales and promote the product. By using visual channels, and merchandising techniques are used to impact the customer’s decision-making process. The assortment is kept current and renewed by rotating the products.
Customer’s attention is held and visual attraction is increased by attractive packaging and branding. In this scenario, a significant amount of data analysis is still ongoing. The merchandising mechanisms sift through the data, gather insights, and create priority sets for the customers while taking seasonality, relevance, and trends into account.
- Lifetime value forecast
Customer lifetime value (CLV) is a measure of a customer’s profit to the business over the course of the full customer-business relationship in the retail industry. Given that revenues are less predictable than costs, special attention is given to them. Direct purchases allow for the creation of both historical and predictive client lifetime prediction approaches.
The CLV models often gather, categorize, and clean data pertaining to customers’ preferences, costs, most recent purchases, and behavior to arrange them into the input. The most recent transactions’ most current data is the foundation for all forecasts. Consequently, the algorithms of a customer’s lifetime inside a single brand are established and examined.
What is Histogram
- Inventories of products based on consumer trends
Sales increase as a result of clients purchasing additional goods in addition to the ones they need. Through up-sell and cross-sell, aids retailers in merchandising improvement and sales growth. Customers purchase goods in groups. For merchandising optimization, revenue growth, and customer retention, retailers must examine the types of goods that are purchased in combination.
Finding out how often people buy is crucial, as is comprehending how different products relate to one another. The frequency of purchases and correlation can be obtained in a very effective and graphic method with the aid of data science approaches.
- Personalized advertising and product recommendations
Recommendation engines are made with the help of data science, and they have shown to be quite useful for merchants as tools for forecasting consumer behaviour. As one of the greatest methods to study client preferences, retailers have started employing recommendation engines.
The recommendation engines’ filtering methods offer insights by taking into account previous client behavior or a number of product attributes. Retailers can enhance sales and create cutting-edge trends by offering advice. Depending on the decisions that clients make, recommendation engines are able to adapt.
Also refer this article: 10 Python Built-in Functions Which You Should Know While Learning Data Science
- Chain-wide supply management and logistics
Advanced mathematics can now be used with the help of data science, giving us the knowledge we need to maximize return on investment in the most effective way. In order to forecast the behavior of machines, people, and even the weather, predictive logistic analytics is used. Predictive analytics are used by businesses to improve their logistical operation, which results in lower mechanical downtime, more effective routes, more customer satisfaction, and higher stock values.
The most effective way to transport goods and assets around is a problem that businesses frequently face. Numerous factors need to be taken into account, including changing demand, human error, traffic, fuel prices, and weather. As a result of technological advancements, the daily data collected is evaluated to produce valuable insights from which decisions with significant effects on the organization can be made.
- Forecasting demand
The practice of demand forecasting involves predicting consumer demand for goods and services. Retailers can maintain the necessary stock levels thanks to information on how demand will vary. The ability to accurately predict demand can increase a company’s competitiveness across all market segments, including manufacturing, supply, and retail.
Neural networks, data mining, and traditional statistical methods are just a few of the analysis tools offered by data science that can be utilized to model customer demand. Times Series Analysis is one of the most effective Data Science strategies for predicting demand since it is regarded as one of the greatest methods for predicting demand.
Also read this article: Data Science Vs Data Analytics
Future of Data Science in Retail
In many facets of human existence, data science is being applied. To improve the shopping experiences for customers, businesses use a variety of data analysis models. This is done so that the marketing strategies and merchandising procedures can be optimized. All transactions, emails, search inquiries, prior purchases, etc. are studied and processed in this respect.
Future iterations of the virtual world might serve as a venue for online shopping, other forms of entertainment, learning, and even team brainstorming sessions. The good news is that a significant portion of the necessary technology has already been developed. Data science professionals apply their techniques to better serve retail customers. Everything is tracked and analyzed so that advertising and product placement can be optimized, including sales, emails, search searches, and past purchases.
The goal of data science in retail, which is based on big data and data science, is to gather and analyze customer data in order to understand customer preferences and spot patterns. To make more informed business judgments, these are essential. Insights into consumer demands can be gained from a deliberate, data-driven examination of retail transactions. It also provides a wealth of information regarding the effectiveness of vendors, goods, and establishments.
What is Box Plot
Conclusion
Finally, the creation and widespread application of data science in the actual world has ushered in a new era. The world as we know it has transformed as a result of the various retail uses of data science. Each year, retail data’s volume, variety, and value significantly increase. To develop data-driven strategies and raise profit margins, the retail sector uses data science. Retail companies can compete, give customers a better shopping experience, and increase revenue thanks to these data science applications. Additionally, as technology advances, data science has a lot more to offer retailers in terms of improvements.
What is Vector, Multiplication & Addition