Descriptive Vs Predictive Vs Prescriptive Analytics
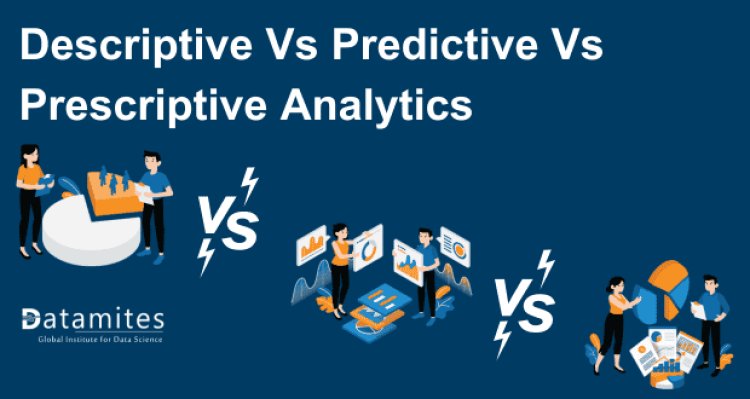
"By 2025, the global market for big data and business analytics is projected to reach $650 billion, highlighting a significant increase in the significance and utilization of data across multiple industries." This staggering statistic underscores the profound impact that data analytics has on modern decision-making. As organizations harness the power of data, they employ various types of analytics to gain insights and drive strategic actions. Understanding the distinctions between descriptive, predictive, and prescriptive analytics is crucial for leveraging data effectively. Let's dive into each type to see how they contribute to the data-driven landscape.
Descriptive Analytics: Understanding the Past
What is Descriptive Analytics?
Descriptive analytics is all about looking back at historical data to figure out what has happened. It’s like reading a detailed report card on past performance. This type of analysis involves summarizing, organizing, and interpreting data to reveal patterns and trends. Essentially, it answers the question, “What happened?” by offering an in-depth examination of historical occurrences and patterns through various methods of data gathering and analysis.
Why is Descriptive Analytics Important?
The primary aim of descriptive analytics is to capture and make sense of historical data. It provides businesses with a thorough overview of their past performance. By analyzing past events, organizations can pinpoint their strengths and weaknesses, identify areas that need improvement, and understand how various factors have influenced their outcomes. This retrospective analysis is invaluable for learning from past experiences and shaping future strategies.
Techniques and Tools: How We Turn Data into Insights
- Data Aggregation: This technique involves gathering data from multiple sources and combining it to create a unified view. Imagine pulling together sales figures from different regions to get a complete picture of your company’s performance across the board.
- Data Mining: This process digs into large datasets to uncover hidden patterns and relationships. It’s like sifting through a massive pile of information to find nuggets of insight that might not be immediately obvious.
- Dashboards: These visual tools present key metrics and trends in a format that’s easy to understand. Dashboards typically utilize charts, graphs, and tables to present data, making it easier to understand intricate information quickly.
- Common Tools: For those diving into descriptive analytics, tools like SQL, Excel, Tableau, and Power BI are often employed. These platforms help in aggregating data, visualizing trends, and generating comprehensive reports.
Real-World Examples: How Descriptive Analytics is Applied
- Sales Reports: Businesses frequently generate monthly or quarterly sales reports that showcase performance metrics, customer behavior, and revenue trends. These reports help in tracking progress and making informed decisions about sales strategies.
- Web Analytics: Analyzing website traffic and user behavior provides insights into how visitors interact with a site. By examining metrics such as page views, bounce rates, and engagement levels, businesses can optimize their online presence and improve user experience.
Benefits and Limitations: What to Consider
Benefits:
- Clear Summaries: Descriptive analytics offers a straightforward summary of what has happened, making it easier to understand past performance.
- Trend Identification: By revealing trends and patterns, it helps in making informed decisions based on historical data.
- Enhanced Understanding: It provides valuable insights into past performance, helping organizations learn from previous experiences.
Limitations:
- Historical Focus: Descriptive analytics only deals with past data and cannot forecast future events or trends.
- Lack of Causation: It may not explain why certain trends occurred, limiting the ability to understand the underlying causes behind observed patterns.
Descriptive analytics is a powerful tool for understanding past performance and trends, but it has its limits. It’s a foundational step that helps organizations grasp what has happened, but for predicting future outcomes and understanding deeper causes, other types of analytics, like predictive or prescriptive analytics, are needed..
Refer these articles:
- Introduction to Data Analytics
- Data Analyst Career Scope
- Exploring Data Analyst Job Opportunities and Salaries Worldwide
Predictive Analytics: Peering into the Future
Predictive analytics is a sophisticated field that delves into historical data and employs advanced statistical algorithms to forecast future events. Predictive analytics revolves around addressing the question, "What might come next?" By analyzing and decoding patterns in historical data, it aims to provide businesses with insights that help them anticipate future developments and trends.
Purpose: Anticipating Outcomes
The core objective of predictive analytics is to equip businesses with the ability to foresee potential developments before they occur. This foresight empowers organizations to take proactive measures instead of merely reacting, thereby securing a competitive advantage. Whether it's understanding future market trends or anticipating customer behaviors, the power of predictive analytics lies in its ability to guide strategic planning and resource allocation.
Techniques and Tools: Crafting Forecasts
To generate accurate forecasts, predictive analytics employs a variety of techniques and tools:
- Machine Learning: These algorithms are designed to learn from data and improve their predictions over time. Machine learning models adapt and refine their predictions as they are exposed to more data, making them increasingly accurate.
- Statistical Modeling: Techniques like regression analysis and time series analysis fall under this category. Regression analysis helps in understanding relationships between variables, while time series analysis is used to predict future values based on previously observed data points.
- Tools: A wide range of tools can assist in conducting predictive analytics.:
- SAS: A suite of software solutions that provides advanced analytics, multivariate analysis, business intelligence, and data management.
- SPSS: A software package used for statistical analysis in social science, offering tools for data management and analytics.
- Python Libraries: Libraries like scikit-learn and TensorFlow are popular for building and deploying machine learning models. Scikit-learn offers a range of tools for data mining and data analysis, while TensorFlow is known for its deep learning capabilities.
Examples: Predictive Analytics in Action
Predictive analytics is applied across various domains to drive decision-making and improve efficiency:
- Credit Scoring: Financial institutions use predictive models to evaluate the creditworthiness of individuals. By analyzing past financial behavior and transaction patterns, these models help in determining the likelihood of a person defaulting on a loan.
- Demand Forecasting: Retailers and manufacturers leverage predictive analytics to forecast upcoming product demand. Accurate demand forecasting helps in optimizing inventory levels, reducing waste, and ensuring that supply chains are well-managed.
Benefits and Limitations: Navigating the Future
Benefits:
- Proactive Decision-Making: Predictive analytics enables organizations to make decisions based on anticipated future scenarios, rather than reacting to events as they happen.
- Resource Optimization: By predicting future needs and trends, businesses can allocate resources more efficiently, enhancing operational effectiveness.
- Enhanced Customer Satisfaction: Understanding customer preferences and predicting their needs helps businesses in tailoring products and services to meet expectations, thereby improving customer satisfaction.
Limitations:
- Data Quality and Skills: To achieve successful predictive analytics, it's essential to have both top-notch data and expert skills. Inaccurate or incomplete data can lead to flawed predictions, while technical expertise is needed to develop and interpret complex models.
- Probabilistic Predictions: The nature of predictive analytics is probabilistic, meaning predictions are based on likelihoods and trends rather than certainties. As such, there is always a margin of error, and outcomes may not always align with predictions.
Predictive analytics is a powerful tool that leverages historical data and statistical models to anticipate future events. While it offers significant benefits in terms of foresight and efficiency, it also comes with challenges related to data quality and the inherent uncertainty of probabilistic forecasts.
Read these articles:
- How to Become A Data Analyst
- What is Datamites Certified Data Analyst Certification
- What would be the Data Analyst Course Fee in India
Prescriptive Analytics: Moving Beyond Prediction
Definition: What Should We Do?
Prescriptive analytics takes the insights gained from predictive analytics and goes a crucial step further. While predictive analytics might tell you what is likely to happen in the future, prescriptive analytics answers the more proactive question: "What should we do about it?" It recommends targeted actions to maximize results by analyzing forecasted trends and data.
Purpose: Driving Better Decisions
The main goal of prescriptive analytics is to offer actionable recommendations that can guide decision-making. By integrating data, advanced algorithms, and established business rules, prescriptive analytics identifies the optimal courses of action. This helps organizations make informed choices that can lead to more efficient and effective results.
Techniques and Tools: How Actions Are Recommended
- Optimization: This involves using mathematical techniques to find the most effective solution from a range of options. For instance, optimization can help determine the best allocation of resources or the most efficient route for delivery trucks.
- Simulation: By modeling complex systems and scenarios, simulation allows businesses to evaluate the potential outcomes of different actions. This helps in understanding how changes in variables might affect the overall system and assists in planning for various future scenarios.
- Decision Support Systems: Tools like IBM’s ILOG CPLEX and simulation software such as AnyLogic are used to support decision-making. These tools apply sophisticated algorithms and simulations to recommend the most effective actions.
Examples: Real-World Applications
- Route Optimization in Logistics: Businesses in logistics use prescriptive analytics to determine the most efficient delivery routes. This optimization reduces transportation costs, enhances delivery speed, and improves overall service quality.
- Personalized Marketing: Companies use prescriptive analytics to craft personalized marketing strategies. By analyzing customer preferences and behaviors, businesses can recommend tailored offers and promotions that are more likely to engage individual customers.
Benefits and Limitations: Implementing the Best Actions
Benefits:
- Clear Recommendations: Prescriptive analytics provides straightforward suggestions for action, making it easier for decision-makers to implement effective strategies.
- Enhanced Decision-Making: By considering various scenarios and constraints, it helps organizations make well-informed decisions that are likely to lead to better outcomes.
- Improved Efficiency: Businesses can optimize their operations, leading to greater efficiency and effectiveness in achieving their goals.
Limitations:
- Complexity: Implementing prescriptive analytics can be challenging and often requires specialized expertise. It involves complex models and a deep understanding of the underlying data.
- Data and Model Dependency: The quality of the recommendations is heavily dependent on the accuracy of the data and the robustness of the models used. Poor data quality or flawed models can lead to suboptimal recommendations.
Prescriptive analytics represents a significant advancement in the realm of data-driven decision-making, offering not just insights but actionable guidance to steer organizations towards their objectives.
Comparing the Three Types of Analytics
1. Descriptive vs. Predictive Analytics
Key Differences:
Descriptive Analytics is all about summarizing past data to gain insights into historical events and trends. It involves techniques like data aggregation, data mining, and reporting to provide insights into past events and trends.
Examples: Monthly sales reports, customer behavior analysis from past data, and historical financial performance.
Predictive analytics leverages past data to project future trends. It employs statistical models and machine learning algorithms to predict future trends or behaviors based on past patterns.
Examples: Sales forecasts, risk assessment models, and customer churn predictions.
How They Complement Each Other:
Descriptive analytics provides the foundational data and insights that predictive analytics builds upon. By understanding historical patterns through descriptive analytics, organizations can create more accurate predictive models.
For instance, if descriptive analytics reveals a pattern in seasonal sales spikes, predictive analytics can use this information to forecast future sales spikes and plan accordingly.
2. Predictive vs. Prescriptive Analytics
Transition from Forecasting to Action:
Predictive Analytics focuses on forecasting what might happen in the future based on historical data and trends. It provides insight into potential future outcomes.
Examples: Predicting customer purchase behavior, identifying potential equipment failures, and estimating market demand.
Prescriptive Analytics goes a step further by recommending actions based on the predictions. It uses optimization algorithms and decision models to suggest the best course of action to achieve desired outcomes. It answers the question, "What should we do about it?"
Examples: Recommending inventory levels to meet predicted demand, suggesting marketing strategies to capitalize on forecasted trends, and advising on risk mitigation strategies.
Integration of Predictive and Prescriptive Analytics:
Predictive analytics identifies potential issues or opportunities, while prescriptive analytics offers actionable recommendations to address them. For example, if predictive analytics forecasts an increase in customer demand, prescriptive analytics can suggest optimal inventory levels and promotional strategies to meet this demand.
3. Descriptive vs. Prescriptive Analytics
Descriptive Data Informing Prescriptive Decisions:
Descriptive Analytics offers a detailed view of historical performance and emerging patterns. This historical perspective is essential for prescriptive analytics to make informed recommendations.
Example: Descriptive analytics might show that certain marketing campaigns were particularly successful in the past. Prescriptive analytics can then recommend similar strategies for future campaigns to maximize success.
By analyzing descriptive data, organizations can identify patterns and trends that serve as a basis for prescriptive recommendations. For instance, if descriptive data shows that customer engagement increases with personalized offers, prescriptive analytics can advise on how to tailor offers for different customer segments.
Factors to Choose the Right Type of Data Analytics
Selecting the right type of data analytics depends on several factors:
Business Needs: Understand your organization's goals and what kind of insights are required to drive decisions. Are you looking to understand past performance, predict future trends, or get actionable recommendations?
Resources and Expertise: Assess the tools, technology, and skills available within your organization. Descriptive analytics might be handled with basic tools and skills, while predictive and prescriptive analytics often require advanced software and specialized expertise.
Use Case Scenarios: Determine which type of analytics best suits your needs:
- Descriptive Analytics: Ideal for summarizing past performance and identifying historical trends.
- Predictive Analytics: Useful for forecasting future outcomes and assessing potential risks.
- Prescriptive Analytics: Best for obtaining actionable recommendations to optimize decision-making.
Understanding the different types of analytics and how they interact can significantly enhance your data strategy. By leveraging descriptive, predictive, and prescriptive analytics, organizations can transform raw data into actionable insights, drive strategic decisions, and gain a competitive advantage. For those looking to master these powerful tools, enrolling in a comprehensive data analytics training program can be a valuable investment in unlocking the full potential of data-driven decision-making.
DataMites is a leading provider of professional training in data science and analytics. DataMites comprehensive Data Analytics Course is designed to equip learners with essential skills in data interpretation and analysis. Partnering with esteemed organizations like IABAC and NASSCOM FutureSkills, DataMites offers industry-recognized certifications. Additionally, DataMites Institute provides a variety of courses, including Artificial Intelligence, Data Science, Machine Learning, and Python Programming. These courses are meticulously crafted to meet the evolving demands of the tech industry, enhancing both career prospects and technical expertise.