Data Science in E-Commerce – Revamping the Shopping Experience
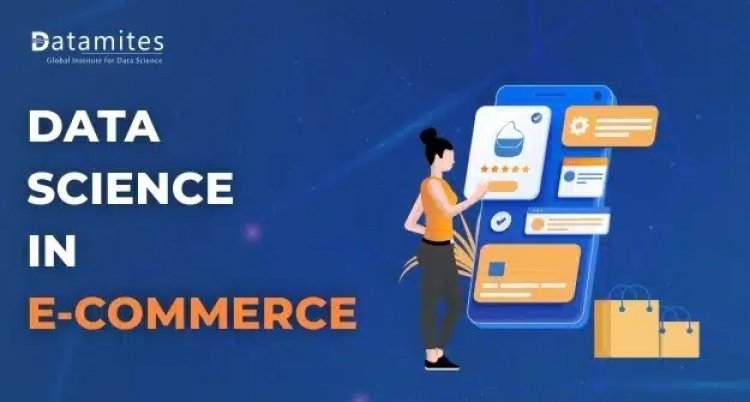
Perchance the growth of e-commerce websites is one element that everyone seems to love in the internet world. Any item, from a pair of gowns to a gadget to a pair of sneakers to some last-minute groceries, may be ordered online and delivered directly to your door. These upsides of modern e-commerce are crazy, obviously who would have thought by the 21st century the world would have evolved as much as it has?
Retail Vs E-Commerce
The notions of retail and e-commerce sometimes overlap. The development of technology has given rise to a number of efficient, secure, and time-saving options, including internet shopping. One of the greatest improvements nowadays is the ability to purchase from the convenience of one’s home throughout the globe. Despite having many similarities, e-commerce and retail operate differently when it comes to trading. Before moving onto the main topic of the article, let’s take a quick tour to distinguish the two.
Retail – refers to the sale of goods in shops, one-on-one, or via mail.
- Possibility of direct product contact.
- Quick access to items.
- Shopping is permitted during store hours.
- Direct communication with staff.
- Time-consuming to find the things you would want.
E-commerce – the sale of goods only online.
- Using images and videos to learn about products in online commerce.
- The delivery of the goods is slow.
- Shopping is accessible 24/7.
- Humans are available during customer care, and chatbots are available 24/7.
- Easily find desired products through search options.
The rise of information technology perhaps brought the biggest change. In fact, e-commerce, a brand-new type of trade, was formed as a result. Although e-commerce hasn’t completely overtaken traditional retail, it has changed how we view shopping and now accounts for a larger portion of overall retail sales than it did even a few years ago. According to Nasdaq Composite, by 2040, it is anticipated that eCommerce would account for 95% of all purchases.
Refer to the article: 10 Python Built-in Functions Which You Should Know While Learning Data Science
Applying Data Science to E-Commerce
Let’s find a quick example to know how data science really does revolutionize the shopping world. In case, you have been a regular Amazon, Flipkart, or Myntra user and searched for a professional suit on each of the websites. Even after you are over with this search and go about with other things in life, you will continue to get product recommendations from these platforms. And how is that?
If these platforms don’t employ a sophisticated data analytics system, how on earth is it going to assess what its millions of users are really too interested to buy? What about the ‘Top Picks’ and the ‘Recommended for You’ options that they provide? Also, the streaming juggernaut, Netflix is currently leveraging that brand familiarity to find success in e-commerce, a new industry. Netflix recently opened an online store where fans of its famous shows may purchase limited-edition goods. Also data science, there!
Let’s now go into depth at some other ways that data science is being used in e-commerce. One at a time.
- Personalized Recommendations Systems
Do you realize that Amazon, Flipkart, or any other e-commerce website gives you a variety of choices regarding the products you wish to buy or are interested in? How can these websites understand what you want, then? They make use of data science’s magic, that’s how!
Recommendation systems keep track of the products you purchase, the pages you click on, the products you are interested in, and other information. It then uses data science to analyze this information and generate recommendations for you based on your profile. As a result, each user of these e-commerce sites would get unique personalized recommendations based on their browsing habits, past purchases, etc.
Data science algorithms understand the many product qualities and relationships as well as how to predict client demands by observing consumer preferences. By altering the gallery pages for a particular client or the order of the products in the search results of the mobile app or website, data science algorithms assist in personalizing the consumer experience. This is a real winner because it practically seems to recommend things that consumers will always want to buy or at the very least show interest in. Creating the correct product and placing it in front of the right customer, results in improved sales.
- Getting Insights on Customers – Retention, Cross-Selling, and Up-Selling
In order for e-commerce firms to survive, collecting customer data has become crucial due to the shifting consumer trends, declining client loyalty, and increasing customer expectations. Companies are routinely on their hunt for new consumers and retain the trust and loyalty of those customers who continue to buy their products and services despite the availability of others on the market.
Aren’t we all familiar with e-commerce websites encouraging us to buy related or complementary products in the category called ‘frequently bought together,’ and persuading us to buy a product that is equivalent to but more expensive than the one being considered to be bought? These are Cross-Selling and Up-Selling techniques respectively, used immensely by eCommerce companies to increase their sales and thereby improve their financial stand. With more sophisticated analytics namely classifiers, segmentation, clustering, predictive modeling, and such, data science techniques prove to be a valuable tool in the industry.
- Analysis of User Response
E-commerce businesses benefit financially from satisfied clients. Thus, unless they want to go out of business, they cannot afford to ignore the feedback from their customers. The majority of businesses fail because they don’t pay enough attention to client input or quickly fix their issues. However, this is easier said than done, particularly for major e-commerce businesses with millions of clients and thousands of products on the market. However, data science can help them in this situation as well.
To comprehend the overall attitude of their customers and determine whether it is positive, negative, or neutral, businesses can utilize natural language processing, computational linguistics, text analysis, etc. If there is a terrible feeling, they can try to figure out what the issue is and work to fix it.
Read this article: Data Science Vs Data Analytics
- Creating a product strategy to get the ideal product mix
E-commerce isn’t an easy task, to begin with. To sell out on their products and services the companies need to be mindful of a variety of things that include; What goods ought they to market? And when and at what price should the products be offered for sale? Companies should make a complete list of what products they need to release at a given season, the quantity element, and more which will in fact have a significant impact on how the sale goes.
Data science algorithms aid in the definition and optimization of the product mix for e-commerce companies. Every e-commerce company has a product team that examines the design process and searches for opportunities for data science algorithms to assist with forecasting all those necessary elements to enable the creation and selling of a satisfactory product.
- Modeling the Supply Chain to Predict Effective Delivery
The proper quantity of goods must be available at the proper time and location for eCommerce enterprises to make sales. Data science algorithms carry out in-depth analysis to create sophisticated prediction models that assist e-commerce organizations in maximizing consumer pleasure, lowering risk, and forming strategies.
- Optimizing Prices for Increased Sales
Cost analysis, market segmentation, competitor analysis, and other factors need to be carefully considered when optimizing prices, one of the most important parts of any organization. Data science techniques for price optimization take into account a variety of variables, including price flexibility, the item’s size, the customer’s attitude, the price they paid, the time of year, and the like.
Machine learning techniques are used to compute everything. Following that, it determines a price that is advantageous to everyone. Retailers may sell their goods effectively and at the best price with this effective tool. In e-commerce, prices are a crucial component. Would you, after all, purchase earphones on Flipkart that you deemed to be too pricey? Or perhaps you decide that Amazon offers a better price on those earphones and purchase them there. In order to ensure that customers would purchase their products, e-commerce companies must ensure that their pricing is both enticing and affordable while still being expensive enough to generate a profit.
Data Science assists e-commerce companies by applying pricing optimization to help them navigate this extremely narrow rope. Algorithms for determining prices take into account a number of factors, including customer purchasing habits, pricing strategies used by competitors, price flexibility, customer geography, etc. E-commerce websites can ascertain the best pricing in this manner.
Also refer this article: What does the Future hold for Data Science?
- Customer Lifetime Value Prediction
For eCommerce businesses, every customer has a lifetime value, or how much profit they will bring in over the course of their relationship. In order to determine the customer lifetime value and comprehend the importance of a customer to their organization, companies might apply data science to their operations.
Data science reviews the customer’s purchases, online pursuits, product preferences, and other website behavior. The business will then be able to identify which clients are below-zero consumers – those who actually cost the business more money than they are worth and which customers make up the best customer categories.
- Consumer Sentiment Analysis
In the business sector, sentiment analysis of customers had been practiced since long ago. But the task was a laborious and time-consuming one when done through the traditional methods. Now that data science has come into the picture, machine learning algorithms support automation, time savings, and simplification while producing accurate results.
The easiest way to analyze how and what the customers are feeling is through social media. Through language analysis, companies are now able to identify the words reflecting consumer sentiment towards the brand and thereby enabling them to create new and innovative products and services that evoke customer interest.
- Evaluating the Warranty of Products
Having an appropriate warranty tag for products is necessary for a business to sustain growth, indeed there are two sides to a coin. In case the warranty is excessively higher, consumers would likely return the products and companies are likely to lose money whereas if the warranty is too short customers would likely receive defective products that are not returnable. This is why it’s crucial to have a warranty period that’s just right, enough for legitimate customers to return their defective products, but not long enough for fraud.
With data science, retailers and manufacturers can verify their items, their potential lifespan, faults, and returns, and even look for fraudulent activities by analyzing warranty data. In order to analyze warranty data, it is necessary to estimate the distribution of failures based on information such as the age and quantity of returns as well as the age and quantity of still-operational units.
Retailers and manufacturers evaluate how many units were sold and how many were returned because of issues after reviewing the data. They concentrate on looking for irregularities, in warranty claims. It’s a great way for retailers to value their warranties and offer them to customers as a package when they buy their products.
- Identifying Fraud
There are significant chances of fraud when anything is totally online. This is true when individuals attempt credit card fraud on e-commerce websites or frequently purchase items only to return them later. However, data science assists these businesses in identifying fraud and dubious client behavior in order to reduce losses. Data analytics can identify and freeze a user account when credit card fraud causes irregularities in financial transactions and credit card history. The usage of clustering algorithms can also be used to identify patterns of questionable behavior, such as buying the same thing again, buying it in quantity, etc. Data science can help in this way.
- Inventory Management
In order to meet client demand, it’s critical for businesses to maintain an inventory of every item they own. If an item appears to be accessible on the website but was actually unavailable, or if the most popular things had low stock levels and those that are never sold out are in bulk, an eCommerce business would be able to run effectively.
It is inevitable that businesses know what products of them are in high demand and are sold out in bulk so as to increase the quantity of producing the following items and managing it in the inventory. Inventory control is the key to successful selling, given that businesses sell so many of their items in bulk, they require effective data science and data analytics algorithms to maintain their inventory.
Also read this article: Role of Data Science in Making Education A Lot Better
- Merchandising
Any retail operation needs to have effective merchandise. The goal is to develop tactics that boost the product’s sales and marketing. Through visual channels, marketing seeks to affect consumer choice. While appealing packaging and branding draw in customers and improve the visual appeal, rotating products keep assortments feeling new and fresh.
The merchandising algorithms scan data sets for insights and create priority lists of customers while taking seasonality, relevance, and trends into account.
Python for Data Science
Data Science in E-Commerce – The Future
Data science in the e-commerce sector is arguably one of the most promising technologies today. Businesses use data science and machine learning technology in e-commerce to improve customer experiences and preserve the steadfast loyalty of website visitors.
By gathering and incorporating data on customer web behavior, life events, what motivated them to make a purchase, how they engage with various channels, etc., data science in eCommerce aids businesses in providing a fuller understanding of their customers. According to reports, 60% of people use different channels, including mobile, social media, in-store, websites, etc., to study and interact with companies.
The E-Commerce market is booming and data science holds the possibility of improving consumer shopping habits, which will benefit e-commerce companies by upgrading their marketing strategies and profitability. Today, E-commerce behemoths have the ability to connect with their customers and give them a tailored experience thanks to data science.
For many eCommerce businesses, data science is unquestionably helping them grow online sales and improve the client shopping experience. Of course, data science has proven to be quite helpful in attracting clients and boosting sales.
What is Histogram
Final Say
Applications and technologies based on artificial intelligence and data science have permeated our society so profoundly that they are now employed in every business, let alone the one that deals with online sales. It’s possible that you aren’t even aware of the use of AI and data science in some situations because they are so smoothly incorporated into the scene.
In the end, it is likely that changes to the way the e-commerce sector functions will persist soon. And eventually, even the ways in which data science is applied in e-commerce will change.
Certified Data Scientist (CDS) Program