Deep Learning vs Machine Learning
Deep Learning vs Machine Learning explores the key differences between these two AI techniques, focusing on their applications, complexities, and data processing methods. While machine learning uses algorithms to make predictions, deep learning leverages neural networks for advanced pattern recognition.
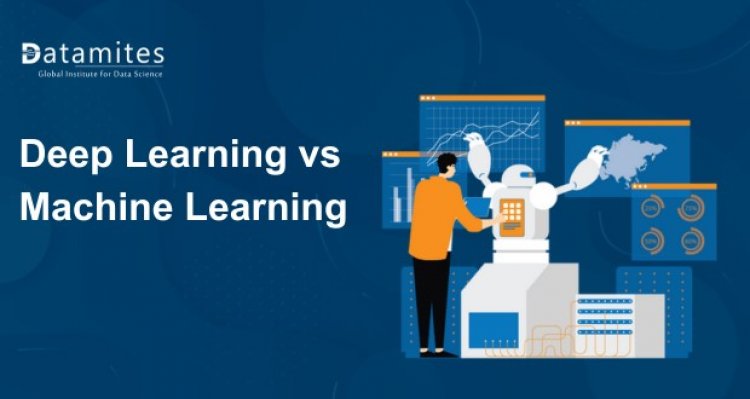
Deep learning and machine learning In the field of artificial intelligence, both of these terms are used interchangeably. So, it’s crucial to understand the main distinctions between machine learning and deep learning. Knowing that deep learning is a subset of machine learning alone makes it simpler to comprehend how machine learning and deep learning compare. And each of these technologies is a subset of artificial intelligence. Artificial intelligence (AI) and the automation of human tasks using AI are hot topics right now. To increase profits, every business seeks to integrate AI into its existing technology. Machine learning and deep learning are two aspects of the vast area of artificial intelligence.
In this article, we will discover the meaning of deep learning as well as machine learning and the differences between these two.
Read These Articles:
What Is Deep Learning?
An artificial neural network that mimics the brain’s neural network is known as deep learning. Several layers are used by the computer to learn from the data. The number of layers in the model serves as a measure of the depth of the model. In terms of Artificial Intelligence, deep learning is the new state-of-the-art. A neural network is used for the learning process in deep learning. The layers of a neural network are piled on top of one another in their architecture.
What Is Machine Learning?
Machine learning is the subfield of AI. A machine using data to “learn” based on it is the fundamental tenet of machine learning. As a result, machine learning systems can swiftly apply knowledge and training from vast data sets to excel at tasks like translating, recognizing individuals, and recognizing voice and objects. Instead of creating and coding a software program with detailed instructions to carry out a task, machine learning (ML) enables a system to learn to identify patterns on its own and make predictions. The goal of machine learning, a particularly practical area of artificial intelligence, is to create computer programs that can automatically learn from past data in order to gather experience and gradually improve their learning abilities in order to make predictions based on fresh data.
Read These Articles:
- How to Become A Data Analyst in India
- How to Become an Artificial Intelligence in India?
- How To Become Data Scientist In India
Deep Learning vs Machine Learning
- Human intervention- Whereas a human must manually choose and code the applied features in machine learning systems based on the type of data, a deep learning system seeks to learn such features on its own. Consider a facial recognition software system. A face’s borders and lines are the first things the program learns to detect and recognize, followed by the face’s more important features and lastly, its overall appearance. The amount of data needed to achieve this is enormous, and the likelihood of getting the correct answer increases as time goes on and the program is improved. As neural networks are used, which are similar to how the human brain works, no human recording of the program is required for this training.
- Time- As you might expect, the massive volumes of data required, the quantity of parameters, and the complex mathematical formulas required to train a deep learning system can make the process time-consuming. Machine learning can be finished in just a few seconds to a few hours, but deep learning can take a few hours to a few weeks.
- Hardware- Due to the volume of data processed and the complexity of the mathematical computations required by the algorithms utilized, deep learning systems require technology that is significantly more powerful than machine learning systems. Graphics processing units are one form of hardware used for deep learning. Machine learning applications can run on less powerful devices with less processing resources.
- Applications- Simple machine learning applications include email spam IDs, predictive algorithms and programs that create evidence-based treatment recommendations for patients. Self-driving cars, which employ numerous layers of neural networks to do tasks like recognizing things to avoid, recognizing traffic lights, and deciding when to speed up or slow down, are one well known application of deep learning. Other examples of deep learning in use include Netflix, music streaming services, and facial recognition.
- Approach- Data is commonly divided into sections by machine learning algorithms, which are then combined to provide a result or solution. Deep learning algorithms take an all-encompassing approach to a scenario or a problem. The problem is solved using a regular process by conventional machine learning methods. It divides the issue into manageable pieces, deals with each separately, and then puts them all together to produce the desired outcome. Instead of segmenting problems, deep learning focuses on solving them from start to finish.
Read These Articles:
Future Of Deep Learning And Machine Learning
Machine learning and deep learning have nearly endless potential uses in the future. Robots will likely spread to other industries and manufacturing, where they can both considerably and marginally improve our daily lives. The healthcare sector will probably also undergo transformation as deep learning enables doctors to perform tasks like predicting or detecting cancer sooner, which can save lives.
For corporations and even for individuals, machine learning and deep learning offer the ability to save costs, make more prudent financial decisions, and allocate resources more efficiently. Additionally, in terms of machine learning and deep learning developments, these three fields represent merely the tip of the iceberg.
Conclusion
Machine learning techniques are used by many AI applications primarily to promote self-service, boost agent productivity, and improve workflows. For many companies and industry titans, machine learning and deep learning algorithms present an exciting opportunity.
DataMites Institute, a renowned center for data science education, offers specialized instruction in subjects like deep learning, machine learning, artificial intelligence, the internet of things, and Python. DataMites’ machine learning courses have been approved by the International Association for Business Analytics Certification (IABAC), an organization with a solid reputation and high regard in the analytics industry.
DataMites Training Institute offers a comprehensive Machine Learning Course, accredited by global certification bodies such as IABAC and NASSCOM FutureSkills. Specializing in AI, Machine Learning, Data Science, Data Analytics, and Python Programming, the institute provides students with hands-on experience through real-time internships, unlimited projects, and access to an exclusive practice lab. This immersive learning experience ensures students are well-prepared to excel in the rapidly evolving tech industry.