Journey from Architect to Machine Learning Engineer – Raaja Vignesh
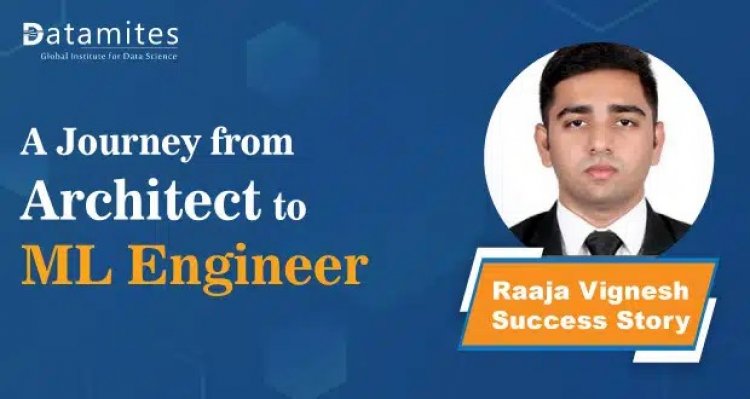
Rarely do we encounter a career journey that goes from architecture to the exciting world of machine learning. What makes someone leave a familiar path and dive into the challenges of data-driven opportunities? Meet Raaja Vignesh, an inspiring example who became a successful Machine Learning Engineer at AccelData, breaking norms and setting his own path.
Amid the stories of career metamorphoses, Raaja Vignesh’s narrative takes center stage – a student at DataMites who skillfully harnessed the power of the institute to reshape his destiny. Is the Transitioning of Careers a Smooth Journey?
Navigating the Shift from Architecture to Data Science: A Daunting Challenge?
Transitioning from a background in architecture to a career in data science can indeed present challenges, as these fields may appear quite different at first glance. However, with the right approach, determination, and guidance, this shift is entirely feasible.
From an architecture enthusiast to a thriving Machine Learning Engineer, Raaja’s evolution symbolizes the art of honing skills in the data science realm. It was within the walls of DataMites that he sculpted his transformation, leveraging their comprehensive resources and expert mentorship to navigate the intricate landscape of data science.
We have interviewed Raaja Vignesh and compiled comprehensive insights into his remarkable journey towards becoming a Machine Learning Engineer. Here we go;
Refer these below articles:
- Artificial Intelligence Vs Machine Learning Vs and Deep Learning
- Difference Between Data Science And Machine Learning
- Machine Learning Engineer vs MLOps Engineer
- What is a Confusion Matrix in Machine Learning?
Q) Good day, Raaja Vignesh. Please tell us a little about yourself.
Hello, my name is Raaja Vignesh, and I am a recent architecture graduate. I am a complete novice in the field of computer science.
Q) What drew you to data science in the first place?
I was in my third year of college, working on digital marketing initiatives and performing some analytical work. In one situation, we were asked to assist a corporation with analytics. This was the turning point in my career when I realized how critical data is in enterprises. As a result, I opted to pursue data science, which is currently the most desired and challenging field on the planet. My passion was not architecture, but rather tinkering with data and solving issues.
Q) How did you come to know about DataMites?
The initial reason I chose DataMites for my data science training was Ashok sir’s profile, which I found to be a fantastic match for me. DataMites reviews on Google and LinkedIn sparked my curiosity about joining the company.
Q) How did your DataMites trainers work out for you?
Ashok sir’s teachings were fantastic; he taught us math and statistics and included case studies in his lectures, which were really beneficial to me. Shubhangi ma’am and Suhail sir were excellent mentors as well. They were able to educate in a way that even a complete newbie like me could comprehend, and there were plenty of opportunities for me to ask questions and get clarification.
Read these below articles:
- A Journey from Fresher to Data Analyst
- Career Transition from a DB Architect to Director Analytics
- How to Get into Data Analytics from a Different Background?
Q) How did the shift from architecture to data science go for you?
Data science is a brand-new discipline, and it doesn’t matter if you’re a novice or a seasoned professional. Start with math and statistics and improve your skills in these areas because they are required for data science and coding. Practice what you’ve learned to improve your knowledge of the subject. I worked on a variety of data sets at DataMites, which helped me tackle a variety of case study-based difficulties. Having a thorough understanding of machine learning could be really beneficial.
Q) Could tell us about your interview process? How did you land your current job?
At AccelData, my first round of interviews consisted of me presenting myself, my background, and my interests, among other things. The interviewer handed me challenges to solve in the second round, which focused on arithmetic and statistics. The final round consisted of a live coding session in which I had to tackle a python programming problem. Finally, I got introduced to the MLOPS team, where we interacted with one another with the primary goal of determining how well I would fit in with the group.
Q) What recommendations would you provide to aspiring candidates?
Never give up hope; if you’re dedicated enough, you’ll be able to make it through data science and land the job. My one piece of advice is to not spend hours learning everything but to set aside enough time to understand the fundamentals, especially in machine learning and statistics. After you’ve finished with the fundamentals, you can go on to the more complex topics. Interviewers primarily assess our core knowledge of these topics, which is why being well-versed in the foundations is critical. Connect with employers on LinkedIn and apply for as many positions as possible. You have a strong connection with the DataMites team, who are extremely helpful to ambitious prospects.
Watch the complete interview here
Bottom Line
The transition from architecture to data science involved not just a change in field, but also a shift in perspective. With the guidance of DataMites, Raaja harnessed his existing analytical skills and diligently acquired new ones to navigate the complexities of data science. The institute’s comprehensive curriculum, industry-recognized certifications, and expert mentorship served as his compass in this uncharted territory.
Through comprehensive training in artificial intelligence, machine learning, data science, python, data analytics courses and more, distinguished mentors, practical applications, the esteemed IABAC Certification, and unwavering commitment to flexibility, DataMites stands as a guiding light, leading aspiring data scientists towards the fulfillment of their professional aspirations in the dynamic terrain of data science.